Econometrics of Inflation
If you think this post is about inflation of the macro variety, you have come to the wrong place!
This is about inflation of the econometric (and typically micro) variety.
When modeling discrete data -- count data or otherwise -- we often find that one value occurs much more frequently than the rest. Standard models for discrete outcomes (usually referred to as limited dependent variable models) do not fit the data well in such situations because they have some type of "smoothness" (implicitly) built in to the assumed data-generating process. This causes the estimated model to under-predict the frequently occurring outcome and over-predict other outcomes.
To better fit the data in such situations, so-called "inflated" limited dependent variable models were created. As far as I know, early work in this area cites Cragg (1971) and Mullahy (1986). The literature started with count data models when zeros occurred with high frequency in the sample. This led to the zero-inflated poisson (ZIP) model, and later the zero-inflated negative binomial (ZINB) model. These models are also referred to as hurdle models and with-zeros models.
I venture to guess that most applied researchers are familiar with the ZIP model. But, if not, I think it is pretty cool. The model assumes the following data-generating process. There are two types of agents, denoted by r=0,1. A type 0 agent always has a count of zero, denoted by y=0. A type 1 agent has a count, y, determined by the usual poisson process (with covariates denoted by x). Thus, observations with y>0 are definitely type 1; observations with y=0 may either be type 0 or 1. Importantly, and interestingly, agent type, r, is unobserved to the researcher. Instead, agent type is determined by a probit model (with covariates denoted by z). The presence of an agent type that always has zero as an outcome inflates the probability of this outcome occurring within the model.
The ZIP model is then estimated by maximum likelihood. The likelihood function is based on the fact that the probability of observing a given value of y depends on both the poisson process and agent type process. As such, we obtain estimates of the coefficients for the poisson process as well as the agent type process. This means we can estimate the determinants of agent type, the probability of a given observation being of each agent type, and the marginal effects of z on the probability of being each agent type. All of this despite not observing agent type in the data!
I remain truly fascinated when we can learn things without even observing the data! But, when you think about, the intuition is so straightforward. The effects are identified because allowing for multiple agent types gives the model another way to explain the presence of zeros. The likelihood function finds the best way to explain the pattern of covariates and outcomes given the two types of processes at work.
Like I said above, most researchers presumably are at least aware of ZIP models. What people are probably less aware of is the tremendous growth in other "inflated" models. Harris and Zhao (2007) propose a straightforward (in hindsight!) zero-inflated ordered probit model. The setup is the same as the ZIP model, except now the outcomes for type 1 agents are modeled using an ordered probit.
Brooks et al. (2012) pivot and propose a middle-inflated ordered probit model. The outcome remains ordered and takes on 3 values, where the middle value occurs with very high frequency. The authors' original application relates to votes on interest rates by the Bank of England Monetary Policy Committee. Uh oh! I promised there would be no macro!
Members can vote to lower interest rates, keep them unchanged, or raise them. Hence, an ordered probit seems potentially appropriate, yet the vast majority of votes are cast for the status quo. In this setup, one can describe a type 0 agent as a member that loves stability/inertia (or is very lazy). Interesting to see who the model predicts is of this type, in addition to providing potentially better insights into the determinants of voting behavior.
I think the zero- and middle-inflated ordered probit models have the potential to be useful to many researchers who may be unaware of their existence. The modeling of lots of outcomes would seem to benefit from some type of inflation factor (if appropriate). There are also other similar models in the literature. Greene and others worked on a more complex model they referred to as a tempered ordered probit. I do not believe it has been published. Extensions to the zero-inflated count models have appeared in the literature, as has a baseline-inflated multinomial logit model in political science.
Perhaps this post will inspire some of you out there to inflate the use of these models!
References
Brooks, R., M.N. Harris, and C. Spencer (2012), "Inflated Ordered Outcomes," Economics Letters, 117: 683-686.
Cragg, J. (1971), "Some Statistical Models for Limited Dependent Variables with Application to the Demand for Durable Goods," Econometrica, 39(5): 829-844.
Harris, M.N. and X. Zhao (2007), "A Zero-Inflated Ordered Probit model, with an Application to Modelling Tobacco Consumption," Journal of Econometrics, 141: 1073-1099.
Mullahy, J. (1986), "Specification and Testing of Some Modified Count Data Models," Journal
of Econometrics, 33(3): 341-365.
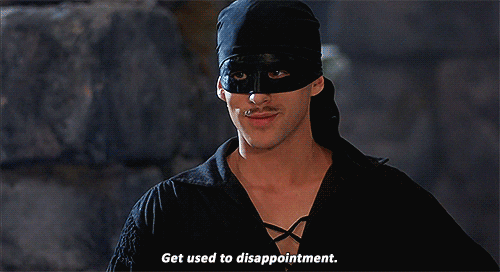
This is about inflation of the econometric (and typically micro) variety.
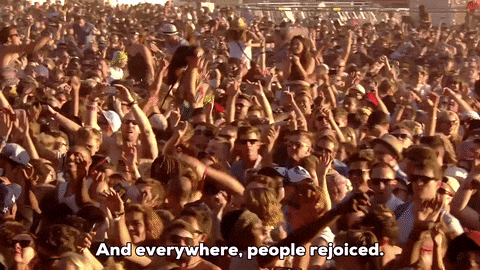
When modeling discrete data -- count data or otherwise -- we often find that one value occurs much more frequently than the rest. Standard models for discrete outcomes (usually referred to as limited dependent variable models) do not fit the data well in such situations because they have some type of "smoothness" (implicitly) built in to the assumed data-generating process. This causes the estimated model to under-predict the frequently occurring outcome and over-predict other outcomes.
To better fit the data in such situations, so-called "inflated" limited dependent variable models were created. As far as I know, early work in this area cites Cragg (1971) and Mullahy (1986). The literature started with count data models when zeros occurred with high frequency in the sample. This led to the zero-inflated poisson (ZIP) model, and later the zero-inflated negative binomial (ZINB) model. These models are also referred to as hurdle models and with-zeros models.
I venture to guess that most applied researchers are familiar with the ZIP model. But, if not, I think it is pretty cool. The model assumes the following data-generating process. There are two types of agents, denoted by r=0,1. A type 0 agent always has a count of zero, denoted by y=0. A type 1 agent has a count, y, determined by the usual poisson process (with covariates denoted by x). Thus, observations with y>0 are definitely type 1; observations with y=0 may either be type 0 or 1. Importantly, and interestingly, agent type, r, is unobserved to the researcher. Instead, agent type is determined by a probit model (with covariates denoted by z). The presence of an agent type that always has zero as an outcome inflates the probability of this outcome occurring within the model.
The ZIP model is then estimated by maximum likelihood. The likelihood function is based on the fact that the probability of observing a given value of y depends on both the poisson process and agent type process. As such, we obtain estimates of the coefficients for the poisson process as well as the agent type process. This means we can estimate the determinants of agent type, the probability of a given observation being of each agent type, and the marginal effects of z on the probability of being each agent type. All of this despite not observing agent type in the data!
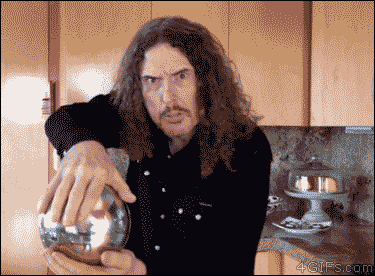
I remain truly fascinated when we can learn things without even observing the data! But, when you think about, the intuition is so straightforward. The effects are identified because allowing for multiple agent types gives the model another way to explain the presence of zeros. The likelihood function finds the best way to explain the pattern of covariates and outcomes given the two types of processes at work.
Like I said above, most researchers presumably are at least aware of ZIP models. What people are probably less aware of is the tremendous growth in other "inflated" models. Harris and Zhao (2007) propose a straightforward (in hindsight!) zero-inflated ordered probit model. The setup is the same as the ZIP model, except now the outcomes for type 1 agents are modeled using an ordered probit.
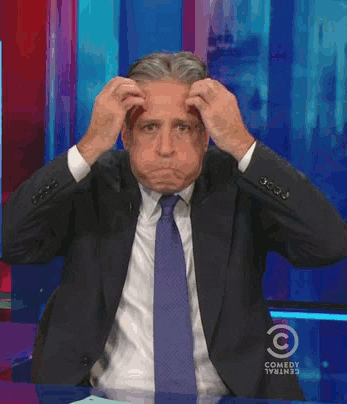
Brooks et al. (2012) pivot and propose a middle-inflated ordered probit model. The outcome remains ordered and takes on 3 values, where the middle value occurs with very high frequency. The authors' original application relates to votes on interest rates by the Bank of England Monetary Policy Committee. Uh oh! I promised there would be no macro!
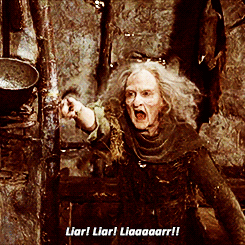
Members can vote to lower interest rates, keep them unchanged, or raise them. Hence, an ordered probit seems potentially appropriate, yet the vast majority of votes are cast for the status quo. In this setup, one can describe a type 0 agent as a member that loves stability/inertia (or is very lazy). Interesting to see who the model predicts is of this type, in addition to providing potentially better insights into the determinants of voting behavior.
I think the zero- and middle-inflated ordered probit models have the potential to be useful to many researchers who may be unaware of their existence. The modeling of lots of outcomes would seem to benefit from some type of inflation factor (if appropriate). There are also other similar models in the literature. Greene and others worked on a more complex model they referred to as a tempered ordered probit. I do not believe it has been published. Extensions to the zero-inflated count models have appeared in the literature, as has a baseline-inflated multinomial logit model in political science.
Perhaps this post will inspire some of you out there to inflate the use of these models!
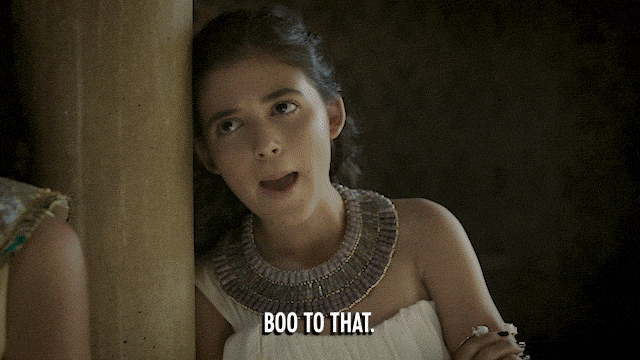
References
Brooks, R., M.N. Harris, and C. Spencer (2012), "Inflated Ordered Outcomes," Economics Letters, 117: 683-686.
Cragg, J. (1971), "Some Statistical Models for Limited Dependent Variables with Application to the Demand for Durable Goods," Econometrica, 39(5): 829-844.
Harris, M.N. and X. Zhao (2007), "A Zero-Inflated Ordered Probit model, with an Application to Modelling Tobacco Consumption," Journal of Econometrics, 141: 1073-1099.
Mullahy, J. (1986), "Specification and Testing of Some Modified Count Data Models," Journal
of Econometrics, 33(3): 341-365.