Time to Dance!
Structural breaks. The stuff of time series. You cannot be a time series econometrician and not be well-versed in the importance of allowing for, testing for, and dealing with structural breaks (or so I am told). However, surely there is something to to be learned from this literature that applied microeconometricians can utilize, no?
Spoiler: Yes, there is!
Applied microeconometricians, who may be unaware of the tremendous advances in the literature on structural breaks, would be wise to take notice. While break dancing may not have advanced since last century and may have little to offer to today's generation, testing for structural breaks has advanced and has much to offer.
y_t = x_t*b2 + e_t, t = T*+1,...,T
Chow (1960) developed a test of the null hypothesis that b1 = b2. Rejection of the null is consistent with a structural break in period T*. While nice and very popular, the Chow Test (as it came to be called) requires the researcher to specify the choice of T*. This is now referred to as testing for the presence of a structural break with a known break date.
Beginning with Andrews (1993), researchers wished to let the data "speak" for itself and inform us if and when a break occurred.
This literature, which continued with Bai (1997), Hansen (2000), among others, is now referred to as testing for the presence of a structural break with an unknown break date. For applied researchers, this advancement in the literature is fantastic; it is informative and it is (relatively) easy to implement. The idea is simply to conduct a grid search over possible break dates and then check for a break in the period with the strongest evidence of a break.
To proceed, define the set of periods where a break may have occurred as [T0,T1], where 1<T0<T1<T. The only constraint on the choice of T0 and T1 is that things are easier if you have sufficient degrees of freedom to estimate the model in the pre- and post-break periods. One sets T* equal to each value in the range [T0,T1] and performs a Chow Test. Thus, one ends up with a collection of T1-T0 Chow test statistics (typically, an F-statistic or a Wald statistic). The T* that maximizes the test statistic is the period where a structural break was most likely to have occurred if there was a break at all. Because T* is the period associated with the largest test statistic, this is referred to as a sup-test (sup for supremum).
To determine if there was a break at this T*, we need to compare the Chow test statistic when T* is used as the break date (i.e., the sup-test statistic) to the appropriate critical value. This critical value is not the usual critical value obtained from a F- or Chi2 distribution since one must account for the multiple testing that arises due to the use of a grid search. Fortunately, this is where the work of Andrews (1993) and others comes in. These critical values are derived for us. If the sup-test statistic exceeds the appropriate critical value, then we conclude that there is statistically meaningful evidence of a structural break and T* is the most likely period for the break.
In time series contexts, structural break testing with an unknown break date can be quite informative as perhaps changes in the DGP may not perfectly align with policy changes or major economic events. These methods can tell us that.
Now, if you have made it this far, I applaud you for sticking it out. Time series is not for everyone; it is an acquired taste for sure. But, that is the exact reason for this post (finally!). I am probably not going out on a limb when I say that most applied microeconometricians are not reading the time series literature on testing for structural breaks.
But, you should! There is nothing limiting the tests for a structural break with an unknown break point to time series contexts, where the point refers to a specific value of a continuous variable reflecting time. Let's re-write the model as
y_i = x_i*b1 + e_i, i є Group 1
y_i = x_i*b2 + e_i, i є Group 2
where Group 1 is defined as those observations with z_i <= Z* and Group 2 is defined as those observations with z_i > Z*. This is the identical setup as above with T* now referred to as Z* (and the t subscripts replaced with i). It should be obvious then that the preceding method can be used to estimate Z* from the data by conducting a grid search over possible values of Z*.
Indeed, this has been done in a microeconomic context that pre-dates the time series econometric work developing the methodology that I cited above. Hotchkiss (1991) builds on the literature describing the different wage determination processes for part- and full-time workers. In her model, let Group 1 denote PT workers, Group 2 denote FT workers, z_i is the usual weekly hours worked by individual i, and Z* is the weekly hours of work that differentiates FT and PT work. Her results are given here (where her H* is my Z*):
Thus, there is interesting heterogeneity over the implied "definition" of FT status across sector. Free research idea: I always thought it would be interesting to re-do her analysis using, say, the PSID or Census to compute Z* year-by-year. Armed with Z*_t, we can then see if how expectations of hours worked have evolved over time in the US labor market. Are workers required to work more over time to continue to be treated as a FT worker? I don't know. Seems interesting to me.
More importantly, though, one might be tempted to conclude from this history that it takes a microeconometrician, Hotchkiss, to advance the time series literature on structural break testing! At least we can say that she (Granger) caused the time series advancements!
The bottom line is this. If, in the course of your empirical micro work, you find yourself arbitrarily splitting your sample using a continuous variable to assess heterogeneity (aka, testing for a structural break), consider taking a methodical approach and letting the data speak for itself.
References
Andrews, D.W.K. (1993), "Tests for Parameter Instability and Structural Change With Unknown Change Point," Econometrica, 61(4):821-856
Bai, J. (1997), "Estimation of a Change Point in Multiple Regression Models," Review of Economics and Statistics, 79(4):551-563
Chow, G.C. (1960), "Tests of Equality Between Sets of Coefficients in Two Linear Regressions," Econometrica, 28(3):591-605
Hansen, B.E. (2000), "Sample Splitting and Threshold Estimation," Econometrica, 68(3):575-603
Hotchkiss, J. (1991), "The Definition of Part-Time Employment: A Switching Regression Model with Unknown Sample Selection," International Economic Review, 32(4):899-917
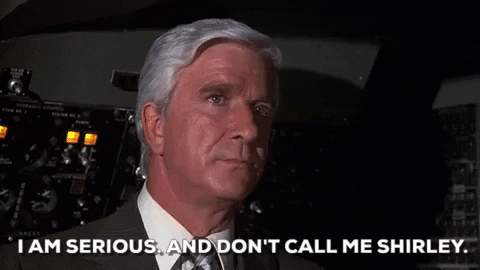
Spoiler: Yes, there is!
Applied microeconometricians, who may be unaware of the tremendous advances in the literature on structural breaks, would be wise to take notice. While break dancing may not have advanced since last century and may have little to offer to today's generation, testing for structural breaks has advanced and has much to offer.

To understand, let us review. A structural break refers to any change in the underlying data-generating process (DGP). Some fraction of the sample is drawn from one DGP; some other fraction is drawn from another DGP. Of course, there may be more than one structural break and, hence, more than two DGPs, but two is sufficient for expository purposes.
In a linear (in parameters) regression set-up, the most common way to think of a structural break is in terms of a change in parameter values. With time series data on y_t and x_t, t = 1,...,T, one might suspect that the data are generated from the following setup:
y_t = x_t*b1 + e_t, t = 1,...,T*
Chow (1960) developed a test of the null hypothesis that b1 = b2. Rejection of the null is consistent with a structural break in period T*. While nice and very popular, the Chow Test (as it came to be called) requires the researcher to specify the choice of T*. This is now referred to as testing for the presence of a structural break with a known break date.
Beginning with Andrews (1993), researchers wished to let the data "speak" for itself and inform us if and when a break occurred.
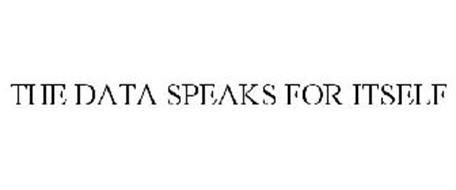
This literature, which continued with Bai (1997), Hansen (2000), among others, is now referred to as testing for the presence of a structural break with an unknown break date. For applied researchers, this advancement in the literature is fantastic; it is informative and it is (relatively) easy to implement. The idea is simply to conduct a grid search over possible break dates and then check for a break in the period with the strongest evidence of a break.
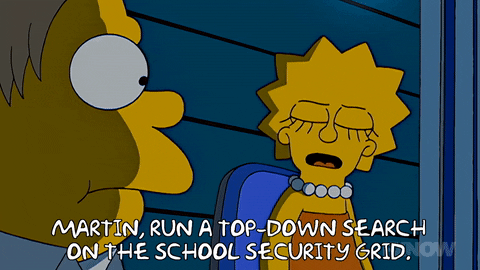
To proceed, define the set of periods where a break may have occurred as [T0,T1], where 1<T0<T1<T. The only constraint on the choice of T0 and T1 is that things are easier if you have sufficient degrees of freedom to estimate the model in the pre- and post-break periods. One sets T* equal to each value in the range [T0,T1] and performs a Chow Test. Thus, one ends up with a collection of T1-T0 Chow test statistics (typically, an F-statistic or a Wald statistic). The T* that maximizes the test statistic is the period where a structural break was most likely to have occurred if there was a break at all. Because T* is the period associated with the largest test statistic, this is referred to as a sup-test (sup for supremum).
To determine if there was a break at this T*, we need to compare the Chow test statistic when T* is used as the break date (i.e., the sup-test statistic) to the appropriate critical value. This critical value is not the usual critical value obtained from a F- or Chi2 distribution since one must account for the multiple testing that arises due to the use of a grid search. Fortunately, this is where the work of Andrews (1993) and others comes in. These critical values are derived for us. If the sup-test statistic exceeds the appropriate critical value, then we conclude that there is statistically meaningful evidence of a structural break and T* is the most likely period for the break.
In time series contexts, structural break testing with an unknown break date can be quite informative as perhaps changes in the DGP may not perfectly align with policy changes or major economic events. These methods can tell us that.
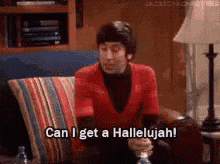
Now, if you have made it this far, I applaud you for sticking it out. Time series is not for everyone; it is an acquired taste for sure. But, that is the exact reason for this post (finally!). I am probably not going out on a limb when I say that most applied microeconometricians are not reading the time series literature on testing for structural breaks.
But, you should! There is nothing limiting the tests for a structural break with an unknown break point to time series contexts, where the point refers to a specific value of a continuous variable reflecting time. Let's re-write the model as
y_i = x_i*b1 + e_i, i є Group 1
where Group 1 is defined as those observations with z_i <= Z* and Group 2 is defined as those observations with z_i > Z*. This is the identical setup as above with T* now referred to as Z* (and the t subscripts replaced with i). It should be obvious then that the preceding method can be used to estimate Z* from the data by conducting a grid search over possible values of Z*.
Indeed, this has been done in a microeconomic context that pre-dates the time series econometric work developing the methodology that I cited above. Hotchkiss (1991) builds on the literature describing the different wage determination processes for part- and full-time workers. In her model, let Group 1 denote PT workers, Group 2 denote FT workers, z_i is the usual weekly hours worked by individual i, and Z* is the weekly hours of work that differentiates FT and PT work. Her results are given here (where her H* is my Z*):
Thus, there is interesting heterogeneity over the implied "definition" of FT status across sector. Free research idea: I always thought it would be interesting to re-do her analysis using, say, the PSID or Census to compute Z* year-by-year. Armed with Z*_t, we can then see if how expectations of hours worked have evolved over time in the US labor market. Are workers required to work more over time to continue to be treated as a FT worker? I don't know. Seems interesting to me.
More importantly, though, one might be tempted to conclude from this history that it takes a microeconometrician, Hotchkiss, to advance the time series literature on structural break testing! At least we can say that she (Granger) caused the time series advancements!
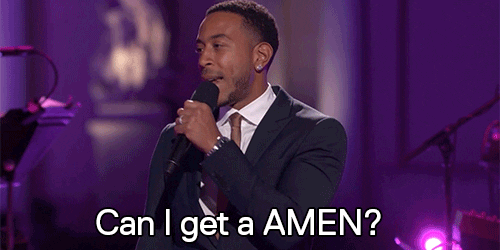
The bottom line is this. If, in the course of your empirical micro work, you find yourself arbitrarily splitting your sample using a continuous variable to assess heterogeneity (aka, testing for a structural break), consider taking a methodical approach and letting the data speak for itself.
References
Andrews, D.W.K. (1993), "Tests for Parameter Instability and Structural Change With Unknown Change Point," Econometrica, 61(4):821-856
Bai, J. (1997), "Estimation of a Change Point in Multiple Regression Models," Review of Economics and Statistics, 79(4):551-563
Chow, G.C. (1960), "Tests of Equality Between Sets of Coefficients in Two Linear Regressions," Econometrica, 28(3):591-605
Hansen, B.E. (2000), "Sample Splitting and Threshold Estimation," Econometrica, 68(3):575-603
Hotchkiss, J. (1991), "The Definition of Part-Time Employment: A Switching Regression Model with Unknown Sample Selection," International Economic Review, 32(4):899-917