Tests for the Rest of Us!
It's not Christmas and, besides, I'm Jewish. But, we could all use a little holiday reference about now. So, let me tell about how I was reminded of this little guy while teaching econometrics this past week, and again while handling a submission as a journal co-editor.
You are probably wondering what Rudolph the Red-Nosed Reindeer has to do with econometrics. Of course, there are a lot of "R"s in his name, but I prefer Stata. So, that's not it. No, I was thinking about poor Rudolph because no one wanted to play any reindeer games with him. In applied econometric research, there are lots of things we could be playing with, but refuse. And, like Rudolph, I do not understand why.
The things I am referring to are model specification tests. This is by no means an area in which I am expert. But there are two simple specification tests that are applicable to probably the vast majority of empirical (micro) research, yet virtually never make an appearance. Like Rudolph, they get left out of all the fun games researchers play with data. Or, like unwanted play things, these tests have seemingly been banished, like those banished to the Island of Mis-Fit Toys.
What tests am I referring to specifically?
The first is Ramsey's (1969) RESET test. RESET stands for Regression Equation Specification Error Test. It is sometimes interpreted as a general test of model specification in a linear regression model. More specifically, it is a test of neglected nonlinearities of the included covariates in the model.
The test is straightforward:
1. Estimate your proposed linear regression model (typically OLS).
2. Form fitted values of the outcome, y-hat.
3. Estimate your proposed linear regression model, augmenting the set of covariates to include y-hat^2, y-hat^3, etc. (typically OLS).
4. Conduct a joint test of the statistical significance of the coefficients on the higher order terms of the fitted values.
There is no firm rule on how many higher order terms to include. The default in Stata is to include 3 terms.
If one rejects the null of zero coefficients, then this suggests you have omitted some important higher order terms of the original covariates, x, or interaction terms between the x's. This is because the higher order fitted values are just (x*beta-hat)^2, (x*beta-hat)^3, etc. and so are functions of higher order terms and interactions involving the x's.
Simple to do, yet the test gets at the fundamental assumption in any (parametric) linear regression model: functional form. And, despite never knowing the correct functional form, Ramsey (1969) has fewer than 3400 citations on Google Scholar. Plus, it was a chapter of his dissertation!
The test is not perfect, I am sure. It is non-constructive in that it does not point to a precisely specified 'true' model if the null is rejected. It may lack some power. But, that just means that failure to reject the null should not be interpreted as evidence that your functional form is correct. Rejection still conveys a lot of useful information.
The second test I am referring to is a test of the strict exogeneity assumption required in fixed (and random) effects panel data models. If you use such models and are not familiar with this assumption, then you should definitely check out this post. It is a very strong assumption and it probably does not hold more often than researchers care to admit.
Again, there is an easy test of this assumption. Augment the model with leads (i.e., future values) of the covariates, x, and estimate the model using the preferred panel data estimator. Test the null that the joint effects of the future covariates are zero. If the model is correctly specified and the covariates are strictly exogenous, future values should not affect current outcomes and the null should not be rejected.
As with the RESET test, the test is not perfect. It is non-constructive in that it does not point to a precisely specified 'true' model if the null is rejected. And, strict exogeneity is only one reason one may reject the null. Nonetheless, rejection still conveys a lot of useful information.
I hope the reason for these tests being overlooked by many empirical researchers is a lack of awareness, and not a lack of desire to know the 'truth' about their model.
Yes, specification testing can be a lot like inviting Scrooge over to your holiday meal. But, tests such as these, or others, should become more commonplace, just as placebo tests have become when available. In an era where the spotlight on replication is getting brighter, we should not leave available tools to combat erroneous research claims on the sideline. They should be allowed to come play with the rest of us!
And let everyday be Festivus!
References
Ramsey, J. B. (1969), "Tests for Specification Errors in Classical Linear Least Squares Regression Analysis," Journal of the Royal Statistical Society, Series B, 31, 350–37
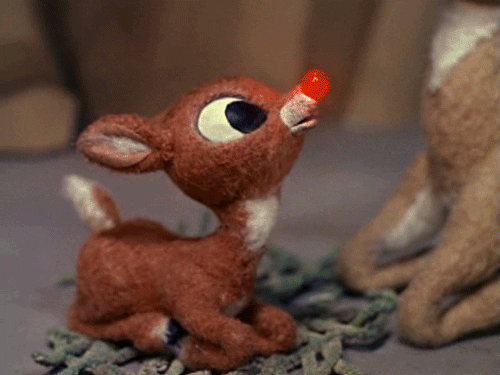
You are probably wondering what Rudolph the Red-Nosed Reindeer has to do with econometrics. Of course, there are a lot of "R"s in his name, but I prefer Stata. So, that's not it. No, I was thinking about poor Rudolph because no one wanted to play any reindeer games with him. In applied econometric research, there are lots of things we could be playing with, but refuse. And, like Rudolph, I do not understand why.
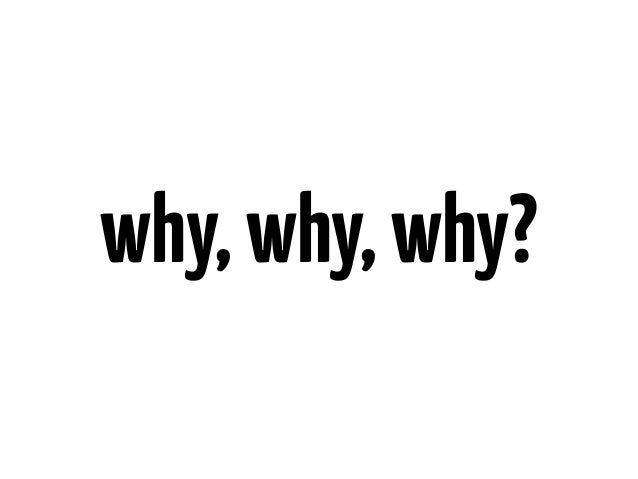
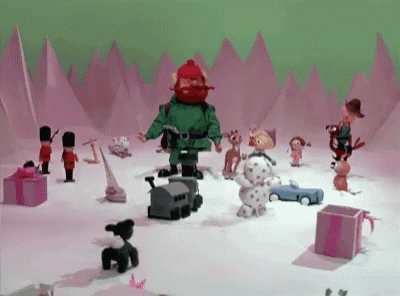
What tests am I referring to specifically?
The first is Ramsey's (1969) RESET test. RESET stands for Regression Equation Specification Error Test. It is sometimes interpreted as a general test of model specification in a linear regression model. More specifically, it is a test of neglected nonlinearities of the included covariates in the model.
The test is straightforward:
1. Estimate your proposed linear regression model (typically OLS).
2. Form fitted values of the outcome, y-hat.
3. Estimate your proposed linear regression model, augmenting the set of covariates to include y-hat^2, y-hat^3, etc. (typically OLS).
4. Conduct a joint test of the statistical significance of the coefficients on the higher order terms of the fitted values.
There is no firm rule on how many higher order terms to include. The default in Stata is to include 3 terms.
If one rejects the null of zero coefficients, then this suggests you have omitted some important higher order terms of the original covariates, x, or interaction terms between the x's. This is because the higher order fitted values are just (x*beta-hat)^2, (x*beta-hat)^3, etc. and so are functions of higher order terms and interactions involving the x's.
Simple to do, yet the test gets at the fundamental assumption in any (parametric) linear regression model: functional form. And, despite never knowing the correct functional form, Ramsey (1969) has fewer than 3400 citations on Google Scholar. Plus, it was a chapter of his dissertation!

The test is not perfect, I am sure. It is non-constructive in that it does not point to a precisely specified 'true' model if the null is rejected. It may lack some power. But, that just means that failure to reject the null should not be interpreted as evidence that your functional form is correct. Rejection still conveys a lot of useful information.
The second test I am referring to is a test of the strict exogeneity assumption required in fixed (and random) effects panel data models. If you use such models and are not familiar with this assumption, then you should definitely check out this post. It is a very strong assumption and it probably does not hold more often than researchers care to admit.
Again, there is an easy test of this assumption. Augment the model with leads (i.e., future values) of the covariates, x, and estimate the model using the preferred panel data estimator. Test the null that the joint effects of the future covariates are zero. If the model is correctly specified and the covariates are strictly exogenous, future values should not affect current outcomes and the null should not be rejected.
As with the RESET test, the test is not perfect. It is non-constructive in that it does not point to a precisely specified 'true' model if the null is rejected. And, strict exogeneity is only one reason one may reject the null. Nonetheless, rejection still conveys a lot of useful information.
I hope the reason for these tests being overlooked by many empirical researchers is a lack of awareness, and not a lack of desire to know the 'truth' about their model.
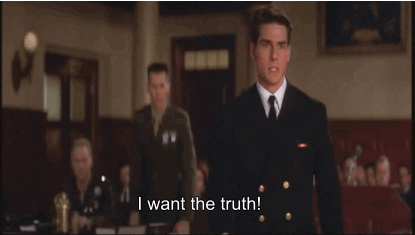
Yes, specification testing can be a lot like inviting Scrooge over to your holiday meal. But, tests such as these, or others, should become more commonplace, just as placebo tests have become when available. In an era where the spotlight on replication is getting brighter, we should not leave available tools to combat erroneous research claims on the sideline. They should be allowed to come play with the rest of us!

And let everyday be Festivus!
Ramsey, J. B. (1969), "Tests for Specification Errors in Classical Linear Least Squares Regression Analysis," Journal of the Royal Statistical Society, Series B, 31, 350–37