Up is Down
It is perhaps fitting that the name of the current pandemic, COVID-19, contains "ID" in its name. It has illuminated a number of statistical issues that researchers have grappled with for decades, if not centuries. But, perhaps the most fundamental issue it has brought to the forefront is identification. In particular, identification of the current infection rate as well as identification of the disease's mortality rate.
As I am sure has been articulated better elsewhere, both of these rates are impossible to identify right now without making heroic assumptions about selection into testing, availability of testing, share of asymptomatic cases, ...
{Y(0), Y(1), D, δ},
but now the following is observed
{y, sign(δ)}.
{Y(0), Y(1), D, δ},
where Y(0) and Y(1) are binary indicators of cold/cough/fever/respiratory symptoms, D is a binary indicator of having COVID-19, and δ is the effect of the disease. Seems obviously reasonable to assume that δ≥0. But, δ is not necessarily one because agents without COVID-19 might have a cold or the flu, and agents with COVID-19 may be asymptomatic. Given that we observe {y, sign(δ)}, can we use this to partially identify Pr(D=1)?
As a second example, we might be interested in saying something about agents who are practicing social distancing. Now, each agent in the population is characterized by the following quantities
{Y(0), Y(1), D, δ},
where Y(0) and Y(1) are binary indicators of COVID-19 infection, D is a binary indicator of practicing social distancing, and δ is the effect of the social distancing. Again, seems reasonable to assume that δ≥0. Given that we observe {y, sign(δ)}, can we use this to partially identify Pr(D=1), the compliance rate with the social distancing recommendations?
I have not worked through this further. Frankly, I am short on mental bandwidth. But, one could start with the Manski (1990) decomposition of the ATE.
Manski, C.F. (1990), "Nonparametric Bounds on Treatment Effects," American Economic Review, 80, 319-323
As I am sure has been articulated better elsewhere, both of these rates are impossible to identify right now without making heroic assumptions about selection into testing, availability of testing, share of asymptomatic cases, ...
When point identification is (near) impossible without leaps of "incredible certitude," partial identification approaches scream for attention (Manski 2011). See my previous post here.
Thinking about what this might entail in the current situation brought to mind an old working paper of mine that was never published. I was then reminded of this again yesterday thanks to a thread on Twitter by Vitor Possebom about a working paper by Jun & Lee (2019).
My old working paper (with John List and Michael Price) was concerned with estimating collusion. Thinking about that problem more than a decade ago, it struck me at the time that one way to proceed was to let up be down and down be up. To turn the objective of causal inference on its head.
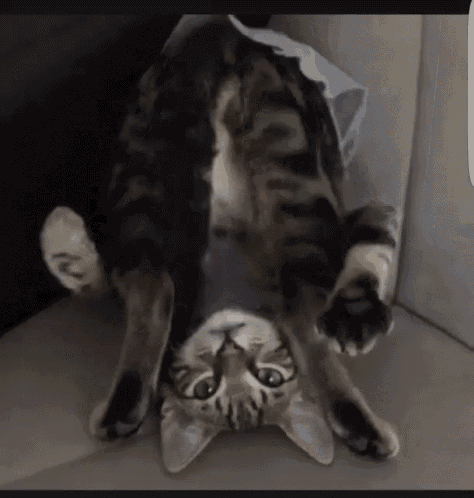
Let's think about the usual setup of the potential outcomes framework and the associated causal inference objective. In this framework, each agent in the population is characterized by the following quantities
{Y(0), Y(1), D, δ}
where Y(0) and Y(1) are the potential outcomes associated with binary treatment, D, and δ is the agent-specific treatment effect, Y(1) - Y(0). Obviously including δ is redundant, but I do say to make it abundantly clear.
For each agent in the econometrician's sample, the following is observed
{y, D}
where y = DY(1) + (1-D)Y(0) is the realized outcome for the agent. As we know, the objective is to overcome the fundamental problem of causal inference and identify δ (or aspects of its distribution such as the ATE) given the data at hand (Holland 1986).
The point is to have this same setup, but vary what we, as the econometrician, know as well as vary our objective. Assume each agent in the population continues to be characterized by the following quantities
{Y(0), Y(1), D, δ},
but now the following is observed
{y, sign(δ)}.
In other words, we know the sign of the treatment effect for each agent, but we do not observe D anymore. And, now, our objective is to say something about D.
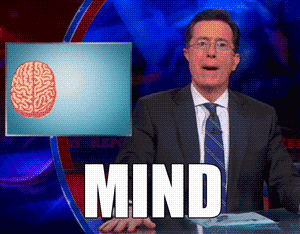
This setup made sense to me in the context of collusion. If bidders in an auction collude, that seems like a binary treatment. And, it seems fairly innocuous to assume that the effect of colluding is to reduce an agent's bid on an object. Knowing this, and seeing all agents' observed bids, y, can we say anything about D? That's what we tried to do in the paper; perhaps not too successfully.
Rethinking about this problem now, we need to start by defining what is our objective. What do we want to say about D? I see three options.
1. Most ambitious: estimate each agent's D, D_i ∀i.
2. Less ambitious: estimate the fraction of the sample that is treated, Pr(D=1)
3. Least ambitious: partially identify the fraction of the sample that is treated, Pr(D=1) ∈ Ω ⊆ [0,1].
In my paper from long ago, when I was young and times were simpler, we focused on 1 and 2. I was foolish. Today, I would focus on 3.
Before thinking about 3 more carefully, the setup here also reminds me of the well-known airport conundrum in the movie masterpiece When Harry Met Sally.
Harry Burns:
You take someone to the airport, its clearly the beginning of the relationship. That's why I have never taken anyone to the airport at the beginning of a relationship.
You take someone to the airport, its clearly the beginning of the relationship. That's why I have never taken anyone to the airport at the beginning of a relationship.
Sally Albright:
Why?
Why?
Harry Burns:
Because eventually things move on and you don't take someone to the airport and I never wanted anyone to say to me, How come you never take me to the airport anymore?
Because eventually things move on and you don't take someone to the airport and I never wanted anyone to say to me, How come you never take me to the airport anymore?
Think about defining a treatment, D, equal to 1 if Harry loves his partner and 0 if he doesn't. Y(0) and Y(1) are binary measures for whether Harry drives his partner to the airport. Harry's partner sees the realized y and is trying to infer D. Up is down. Down is up. Harry Burns style. Of course, Sally's response was ...

And, Vitor's thread about the paper by Jun & Lee (2019) reminded me of this as well. In the paper, the authors are interested in the partial identification of Pr[Y(1)=0|Y(0)=1], where the potential outcomes are binary. One scenario they consider is when the econometrician only observes {y,z} for each agent, where z is an instrument for D. This is similar to my set up of the problem of detecting collusion, except the authors consider the case of an observed instrument, z, correlated with missing treatment status, whereas we considered the sign of the treatment effect as an additional piece of information.
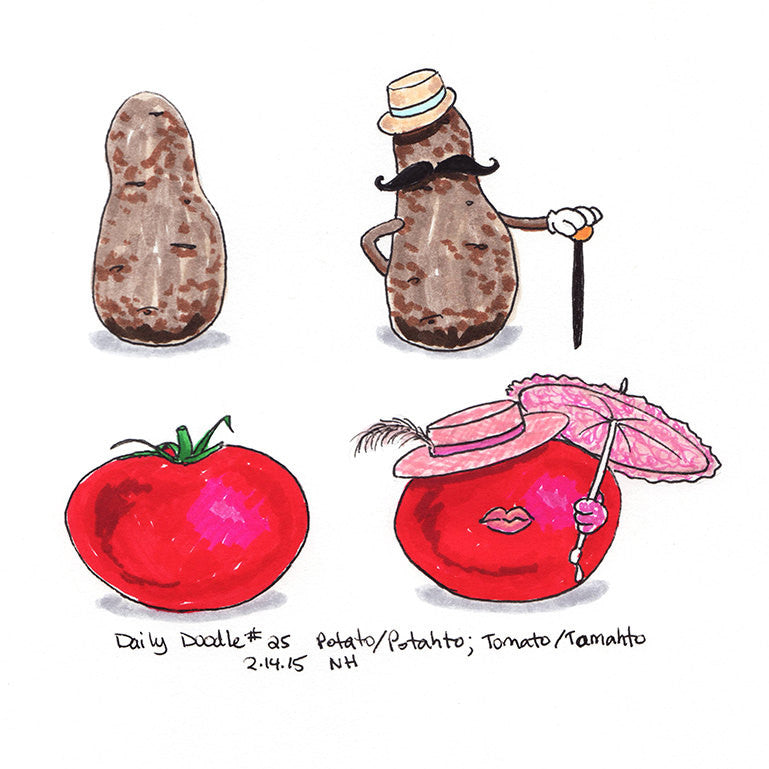
So, returning to ugly reality, I started by talking about the pandemic that is consuming us. How does this apply to that? Well, first let us start with the infection rate of COVID-19. Each agent in the population is characterized by the following quantities
{Y(0), Y(1), D, δ},
where Y(0) and Y(1) are binary indicators of cold/cough/fever/respiratory symptoms, D is a binary indicator of having COVID-19, and δ is the effect of the disease. Seems obviously reasonable to assume that δ≥0. But, δ is not necessarily one because agents without COVID-19 might have a cold or the flu, and agents with COVID-19 may be asymptomatic. Given that we observe {y, sign(δ)}, can we use this to partially identify Pr(D=1)?
As a second example, we might be interested in saying something about agents who are practicing social distancing. Now, each agent in the population is characterized by the following quantities
{Y(0), Y(1), D, δ},
where Y(0) and Y(1) are binary indicators of COVID-19 infection, D is a binary indicator of practicing social distancing, and δ is the effect of the social distancing. Again, seems reasonable to assume that δ≥0. Given that we observe {y, sign(δ)}, can we use this to partially identify Pr(D=1), the compliance rate with the social distancing recommendations?
I have not worked through this further. Frankly, I am short on mental bandwidth. But, one could start with the Manski (1990) decomposition of the ATE.
Here, with the sign of the ATE known, we can figure out what we know or are willing to assume about the terms involving expectations, none of which have observed sample counterparts since D is unobserved, and then solve for the corresponding bounds on Pr(D=1|x) or Pr(D=1). Or, as suggested by Vitor via Twitter, one could combine the fact that
Pr(D=1) = Pr(D=1|y=1)Pr(y=1) + Pr(D=1|y=0)Pr(y=0)
with (partial) information on some of the quantities on the right hand side.
Pr(D=1) = Pr(D=1|y=1)Pr(y=1) + Pr(D=1|y=0)Pr(y=0)
with (partial) information on some of the quantities on the right hand side.
So, while your world is turned upside down due to COVID-19, perhaps turn the causal inference world upside down as well and see where it leads. In the movies, it usually leads to happily ever after.
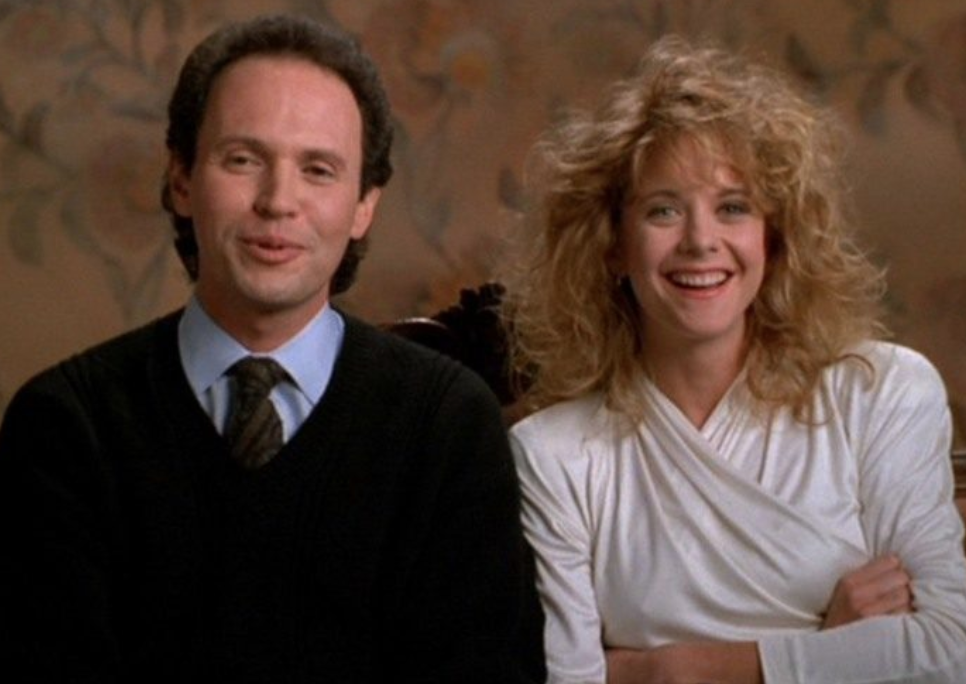
Let's hope it does here as well.
References
Holland, P. (1986), "Statistics and Causal Influence," Journal of the American Statistical Association, 81, 945-960
Jun, S.J. and S. Lee (2019), "Identifying the Effect of Persuasion," https://arxiv.org/abs/1812.02276.
Manski, C.F. (1990), "Nonparametric Bounds on Treatment Effects," American Economic Review, 80, 319-323
Manski, C.F. (2011), "Policy Analysis with Incredible Certitude," Economic Journal, 121, F261-F289.