So Mean
Apparently Taylor Swift was in the news this week. While I can't say I am very up on Taylor Swift songs (or most pop culture for that matter), I did find this line in one of her older songs, Mean, from 2010:
"But you don't know what you don't know"
Seems like very relevant words for empirical researchers. There is so much to know when trying to be a top notch empirical researcher. You have to know the application and all the associated institutional details. You have to know the data and all its quirks (including measurement errors, but that's a topic for another day). And, yes, you have to know the econometrics. But, there is so much on the econometric side, it's hard sometimes for researchers to know what to ask as they don't know what they don't know.
If you are fortunate enough to at least know what you don't know, often times we are afraid to ask. We are afraid of people's response...

Well, sometimes people aren't being mean, but the answer is about the mean. What I mean (pun intended) is the relationship between fixed effects (FE) and correlated random effects (CRE) estimators.
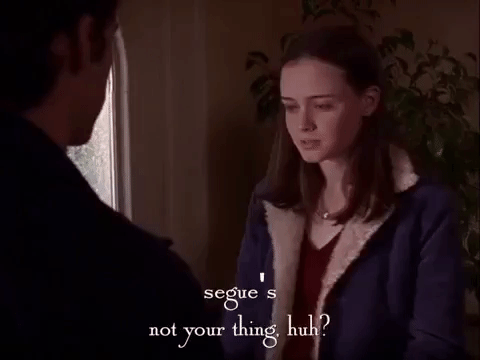
[It's been a loooong week.]
If you are not familiar with CRE, you should be. It's such a simple trick, and so helpful. The idea originates in Mundlak (1978), and then was extended in Chamberlain (1980, 1982, 1984). Mundlak (1978) considers the usual panel data linear regression model with group-specific effects
y_it = a_i + bx_it + e_it
where the time-varying covariates, x_it, are potentially correlated with a_i. In this case, the FE (or "within") estimator provides unbiased and consistent estimates of b assuming that x_it is strictly exogenous (see my previous post).
The FE estimator proceeds by first noting that the model above implies that
ybar_i = a_i + bxbar_i + ebar_i,
where ybar_i, xbar_i, and ebar_i are the group-specific means of y, x, and e, respectively. Subtracting ybar_i from both sides of the original model yields
(y_it - ybar_i) = b(x_it - xbar_i) + (e_it - ebar_i).
An unbiased and consistent estimate of b -- under strict exogeneity -- is obtained by estimating this model by pooled Ordinary Least Squares (POLS). This is the FE estimator.
OK, so many of you presumably know this. Well, along comes Mundlak (1978) and shows that an algebraically identical estimate of b can be obtained by estimating the following
y_it = a_i + bx_it + cxbar_i + u_it
by POLS.
More specifically, this model arises by modeling the dependence between the group-specific effect, a_i, and the covariates, x_it, as
E[a_i | x_it] = cxbar_i.
If we drop the expectation and convert it the error form, we get
a_i = cxbar_i + w_i,
where w_i is mean zero with some variance. Substituting this expression into the original specification,
y_it = a_i + bx_it + e_it,
yields
y_it = bx_it + cxbar_i + w_i + e_it.
Estimating this model using the random effects (RE) estimator produces the identical estimate of b as POLS (only the standard errors will differ). In light of Mundlak's epiphany, RE estimation of the model augmented with xbar_i as additional covariates is known as the CRE estimator of the model.
Prior to continuing, Chamberlain's addition to this literature comes in the form of expressing the dependence between a_i and x_it as
E[a_i | x_it] = c1x_i1 + c2x_i2 + ... + cTx_iT.
Thus, Mundlak's approach is a restricted version of Chamberlain's, and the Chamberlain CRE estimator is the RE estimator applied to
y_it = a_i + bx_it + c1x_i1 + c2x_i2 + ... + cTx_iT + w_i + e_it.
While this is all nice, there is nothing gained here. The Mundlak CRE estimator is just an alternative route to the same spot; the estimator is algebraically identical to the FE estimator.
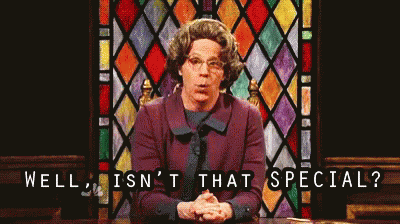
True! But ... only in the context of the linear regression model. In nonlinear models, Mundalk's CRE estimator steals the spotlight.
At the risk of re-starting a culture war, let's think about a binary choice panel data model. In other words, y_it is a dummy variable. With y_it binary and a_i being treated as FEs, a researcher usually considers two options:
1. Ignore the fact that y_it is binary and use the traditional FE estimator discussed above. This is a FE Linear Probability Model (FE-LPM).
2. Estimate a FE logit model.
To say that Option 1 is the popular choice cannot be overstated, but see this awesome tweet this week. Seriously, though, despite its popularity, the FE-LPM has its usual drawbacks.
Option 2 is also not satisfactory. While the FE logit does yield consistent estimates of b assuming the model is correctly specified, with nonlinear models we are no longer interested in b. We are interested in the marginal effects. And, the marginal effects require knowledge of the FEs. Unfortunately, the FE logit proceeds by estimating the conditional likelihood and the FEs drop out. Thus, the only way to recover marginal effects in the FE logit is to add assumptions about a_i which then negates the benefit of the FE model in the first place.
As an aside, for those who may be unaware, there is no FE probit model because there is no way to condition out the FEs from the likelihood. And, failure to condition out the FEs leads to the so-called incidental parameters problem (Neyman & Scott 1948; Lancaster 2000). This incidental parameters problem is what prevents us from simply adding group dummy variables to either the logit or probit model and recovering marginal effects in that way. My simple intuition for the incidental parameters problem is the following. Just as in the linear panel data model with group dummies, the estimates of the FEs are consistent only as T → ∞. So, for small T, the estimated FEs are inconsistent. This inconsistency affects the properties of the estimates of b as well in maximum likelihood (Cameron & Trivedi 2005, p. 781).
Altogether, this means that the FE logit produces consistent estimates of b, but doesn't help us when it comes to marginal effects. A FE probit does not exist (although econometricians continually work on bias-corrected versions). There are even Stata commands! So, for better or worse, empirical researchers revert to the FE-LPM.
But ... Mundlak! Mundlak's approach offers a third option. By modeling the dependence between a_i and x_it as
a_i = cxbar_i + w_i,
one can estimate a RE probit or logit (which do exist and for which marginal effects can be computed ... even in Stata) simply by augmenting the covariate set to include xbar_i (Cameron & Trivedi 2005, p. 786).
So, you see, one doesn't have to resort to a FE-LPM when one wishes to estimate a FE binary choice model. Claims that it is simpler simply are untrue. The same goes for any other non-linear panel data model one may wish to estimate such as count or multinomial models. Sorry to burst your bubble!
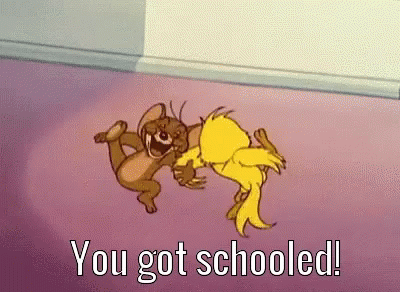
UPDATE (7.27.20)
Thanks to Nicolás Lillo for pointing out a typo on Twitter!
References
Cameron, A.C. and P.K. Trivedi (2005), Microeconometrics: Methods and Applications, Cambridge University Press
Chamberlain, G. (1980), “Analysis of Covariance with Qualitative Data,” Review of Economic
Studies, 47, 225-238
Chamberlain, G. (1982), “Multivariate Regression Models for Panel Data,” Journal of Econometrics, 18, 5-46
Chamberlain, G. (1984), “Panel Data,” in Z. Griliches and M. Intriligator (eds.), Handbook of Econometrics, Volume 2, Amsterdam: North-Holland, 1247-1318
Lancaster, T. (2000), "The Incidental Parameter Problem Since 1948," Journal of Econometrics, 95, 391-413
Mundlak, Y. (1978), "On the Pooling of Time Series and Cross Section Data," Econometrica, 46, 69-85
Neyman, J. and E.L. Scott (1948), “Consistent Estimates Based on Partially Consistent Observations,”
Econometrica, 16, 1–32.