The Average Man
There was a fascinating story posted on Twitter this past week; original source is here. The story concerns a U.S. Air Force pilot, Lt. Gilbert S. Daniels, and how he single handedly saved the USAF in the 1950s. See, pilots were crashing. A lot.
And no one knew why. One thought was that military planes and equipment were ill-sized for USAF pilots in the 1950s as they were built based on specifications of the average USAF pilot in the 1920s. What if the average pilot had changed over the past three decades? In the process of measuring the average specifications of USAF pilots in the 1950s, Daniels made a startling discovery. No pilot is average.
In fact, Daniels found that no pilot was even close to being average. After collecting anthropometric measurements on over 4,000 pilots, he recorded whether each pilot was within the middle 30% of the distribution for each of the ten most salient dimensions for pilots. How many pilots did he find that were close to average within all ten dimensions?
Yup, none! Daniels then wrote up his findings in a 1952 technical report entitled, "The 'Average Man'?". Fascinating. Truly remarkable.
When I read this story, my mind immediately went to econometrics. Not statistics, laws of probability, distributions, etc. To econometrics. Specifically, to marginal effects.
It is presumably well known to most, if not all, reading this that the coefficients in non-linear regression models are no longer of direct interest as these tend to lack a meaningful interpretation. Instead, we focus on marginal effects. Formally, the marginal effect of a particular covariate is the derivative of the conditional expectation function (CEF) with respect to that variable. Note, in a linear regression model (with no interaction or higher order terms), the coefficients are the marginal effects and so there is no distinction.
With non-linear models there is a distinction. Consider a probit model.
Pr(y=1 | x) = Φ(xb)
where Φ is the standard normal cumulative density function (CDF), x are the covariates, and b are the coefficients. The left hand side is equivalent to the CEF for a binary outcome. In this model, each element of b represents the change in the single index, xb, when its corresponding element of x increases by a unit. But, we are not particularly interested in the effect of a change in x on the single index; we care about its effects on the probability that y equals one. This is the marginal effect.
In the probit model, the marginal effect of a unit change in, say, x_1, is
φ(xb)*b_1
where φ is the standard normal probability density function (PDF) and b_1 is the coefficient on x_1.
Yeah, yeah, yeah. The point is that, unlike the coefficient b_1, the marginal effect of x_1 is observation-specific as it is a function of the entire covariate vector, x. This is cool; heterogeneity is real and the model allows for this. However, it does make presenting results a challenge. Nobody wants to see an N x K table of marginal effects. What is one to do?
Conventional practice in economics is to report marginal effects evaluated at the sample mean. Well, Lt. Daniels would surely question the wisdom of this approach.
If we replace x with the vector of sample means, x-bar, then we are potentially reporting the effect of a unit change in each covariate that does not apply to any observation in the real world! This is the equivalent of how the USAF was building planes in the 1950s and the result was a lot of crashing and burning.
As an aside, please indulge me for one moment. Even if one is going to report average marginal effects, there are two non-equivalent ways of doing this. I suspect most researchers are unaware of what is actually being done if they use canned software packages. I know most researchers do not make it clear in their papers which method is being used. The two methods are
1. Compute φ(x-bar*b)*b_k, k=1,...,K
2. Compute φ(x_i*b)*b_k, k=1,...,K, for all i and then compute the mean.
The former is the marginal effect evaluated at the sample mean. The latter is the mean of the observation-specific marginal effects. The -margins- command in Stata performs the latter.
Returning to Lt. Daniels and the current state applied econometric research, what I suspect is that we may have countless studies reporting marginal effects that are of little or no policy relevance. The reported effects of changes in x -- which could be a policy or treatment or intervention -- is for an economic agent that simply does not exist. Dare I say, Hypothetical Homo economicus.
Frankly, this is as scary as countless pilots crashing their planes because their equipment was designed for a hypothetical pilot that does not exist, or at least did not enlist.
So, what can we do?
Fortunately, the solution is fairly trivial, but requires a change in current norms within research. We must change the default approach to quantifying results in applied research and think more carefully about what we chose to report. For example, while not (explicitly) reporting marginal effects, my mind flashed back to this table in Heckman (1974).
Upon estimating a probit model for employment status, he reports the probabilities of employment for different combinations of some covariates, while fixing others at particular values. This makes it explicit to exactly whom the probabilities (and marginal effects, computed as the difference in probabilities across different values of the covariates) apply.
Researchers today should be doing more of the same. I see two options that would improve on the status quo. First, one could amend option #2 from above to
1. Compute φ(x_i*b)*b_k, k=1,...,K, for all i
2. Report specific quantiles of the observation-specific marginal effects such as the 25th, 50th, and 75th quantiles.
If one really wanted to get fancy, one could plot the PDF or CDF of the observation-specific marginal effects. At the very least, the median of the individual-specific marginal effects seems preferable to the mean (if researchers insist on a single column of results in a table) because at least the median is an actual observation. Then, we know that the marginal effects being reported at least apply to someone that exists in the real world. And, if x is a policy chosen by the median voter, then we should at least know the outcome on him/her/they. Alternatively, reporting the interquartile range can be accommodated in a single table column.
Second, one can follow Heckman's lead and report marginal effects for several interesting combinations of hypothetical -- but plausible -- observations.
In hindsight, Daniels' findings reported in "The 'Average Man'?" are probably not that surprising to those well-versed in statistics.
However, we lose sight of its implications when we mindlessly focus on the average in crucial calculations that are made in many studies. A search on google scholar for "marginal effects" reveals more than 34,000 hits since 2010.
Moving forward, let's learn from Lt. Daniels and elevate the quality of our research.
Update
Florian Hollenbach pointed out on twitter that Hanmer & Kalken (2013) discusses this very point! Very cool. Thanks, Florian!
References
Hanmer, M.J. and K.O. Kalkan (2013), "Behind the Curve: Clarifying the Best Approach to Calculating Predicted Probabilities and Marginal Effects from Limited Dependent Variable Models," American Journal of Political Science, 57(1), 263-277
Heckman, J. (1974), "Shadow Prices, Market Wages, and Labor Supply," Econometrica, 42(4), 679-694
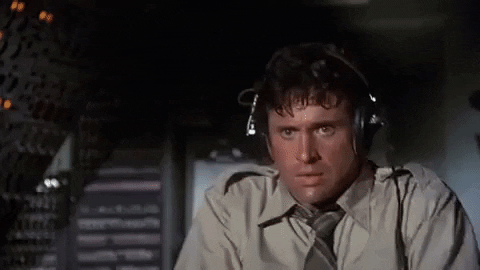
And no one knew why. One thought was that military planes and equipment were ill-sized for USAF pilots in the 1950s as they were built based on specifications of the average USAF pilot in the 1920s. What if the average pilot had changed over the past three decades? In the process of measuring the average specifications of USAF pilots in the 1950s, Daniels made a startling discovery. No pilot is average.
In fact, Daniels found that no pilot was even close to being average. After collecting anthropometric measurements on over 4,000 pilots, he recorded whether each pilot was within the middle 30% of the distribution for each of the ten most salient dimensions for pilots. How many pilots did he find that were close to average within all ten dimensions?
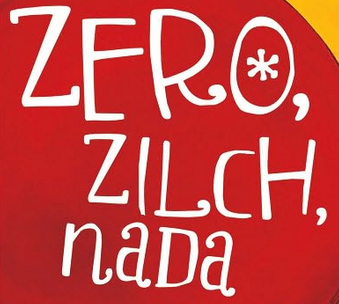
Yup, none! Daniels then wrote up his findings in a 1952 technical report entitled, "The 'Average Man'?". Fascinating. Truly remarkable.
When I read this story, my mind immediately went to econometrics. Not statistics, laws of probability, distributions, etc. To econometrics. Specifically, to marginal effects.
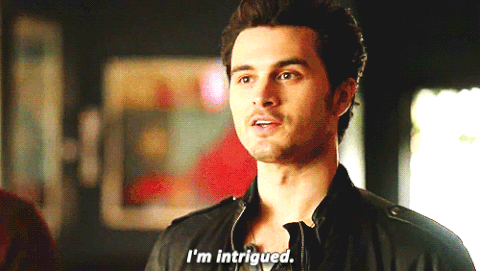
It is presumably well known to most, if not all, reading this that the coefficients in non-linear regression models are no longer of direct interest as these tend to lack a meaningful interpretation. Instead, we focus on marginal effects. Formally, the marginal effect of a particular covariate is the derivative of the conditional expectation function (CEF) with respect to that variable. Note, in a linear regression model (with no interaction or higher order terms), the coefficients are the marginal effects and so there is no distinction.
With non-linear models there is a distinction. Consider a probit model.
Pr(y=1 | x) = Φ(xb)
where Φ is the standard normal cumulative density function (CDF), x are the covariates, and b are the coefficients. The left hand side is equivalent to the CEF for a binary outcome. In this model, each element of b represents the change in the single index, xb, when its corresponding element of x increases by a unit. But, we are not particularly interested in the effect of a change in x on the single index; we care about its effects on the probability that y equals one. This is the marginal effect.
In the probit model, the marginal effect of a unit change in, say, x_1, is
φ(xb)*b_1
where φ is the standard normal probability density function (PDF) and b_1 is the coefficient on x_1.
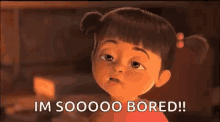
Yeah, yeah, yeah. The point is that, unlike the coefficient b_1, the marginal effect of x_1 is observation-specific as it is a function of the entire covariate vector, x. This is cool; heterogeneity is real and the model allows for this. However, it does make presenting results a challenge. Nobody wants to see an N x K table of marginal effects. What is one to do?
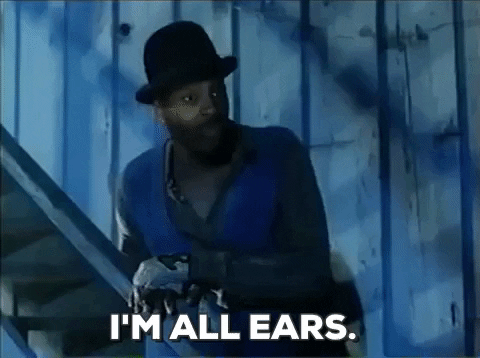
Conventional practice in economics is to report marginal effects evaluated at the sample mean. Well, Lt. Daniels would surely question the wisdom of this approach.
If we replace x with the vector of sample means, x-bar, then we are potentially reporting the effect of a unit change in each covariate that does not apply to any observation in the real world! This is the equivalent of how the USAF was building planes in the 1950s and the result was a lot of crashing and burning.
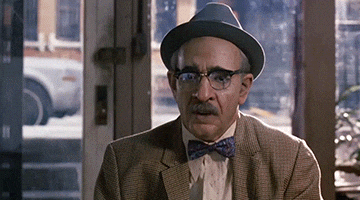
As an aside, please indulge me for one moment. Even if one is going to report average marginal effects, there are two non-equivalent ways of doing this. I suspect most researchers are unaware of what is actually being done if they use canned software packages. I know most researchers do not make it clear in their papers which method is being used. The two methods are
1. Compute φ(x-bar*b)*b_k, k=1,...,K
2. Compute φ(x_i*b)*b_k, k=1,...,K, for all i and then compute the mean.
The former is the marginal effect evaluated at the sample mean. The latter is the mean of the observation-specific marginal effects. The -margins- command in Stata performs the latter.
Returning to Lt. Daniels and the current state applied econometric research, what I suspect is that we may have countless studies reporting marginal effects that are of little or no policy relevance. The reported effects of changes in x -- which could be a policy or treatment or intervention -- is for an economic agent that simply does not exist. Dare I say, Hypothetical Homo economicus.

Frankly, this is as scary as countless pilots crashing their planes because their equipment was designed for a hypothetical pilot that does not exist, or at least did not enlist.
So, what can we do?
Fortunately, the solution is fairly trivial, but requires a change in current norms within research. We must change the default approach to quantifying results in applied research and think more carefully about what we chose to report. For example, while not (explicitly) reporting marginal effects, my mind flashed back to this table in Heckman (1974).
Upon estimating a probit model for employment status, he reports the probabilities of employment for different combinations of some covariates, while fixing others at particular values. This makes it explicit to exactly whom the probabilities (and marginal effects, computed as the difference in probabilities across different values of the covariates) apply.
Researchers today should be doing more of the same. I see two options that would improve on the status quo. First, one could amend option #2 from above to
1. Compute φ(x_i*b)*b_k, k=1,...,K, for all i
2. Report specific quantiles of the observation-specific marginal effects such as the 25th, 50th, and 75th quantiles.
If one really wanted to get fancy, one could plot the PDF or CDF of the observation-specific marginal effects. At the very least, the median of the individual-specific marginal effects seems preferable to the mean (if researchers insist on a single column of results in a table) because at least the median is an actual observation. Then, we know that the marginal effects being reported at least apply to someone that exists in the real world. And, if x is a policy chosen by the median voter, then we should at least know the outcome on him/her/they. Alternatively, reporting the interquartile range can be accommodated in a single table column.
Second, one can follow Heckman's lead and report marginal effects for several interesting combinations of hypothetical -- but plausible -- observations.
In hindsight, Daniels' findings reported in "The 'Average Man'?" are probably not that surprising to those well-versed in statistics.
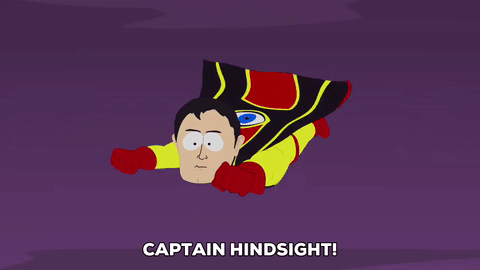
However, we lose sight of its implications when we mindlessly focus on the average in crucial calculations that are made in many studies. A search on google scholar for "marginal effects" reveals more than 34,000 hits since 2010.
Moving forward, let's learn from Lt. Daniels and elevate the quality of our research.
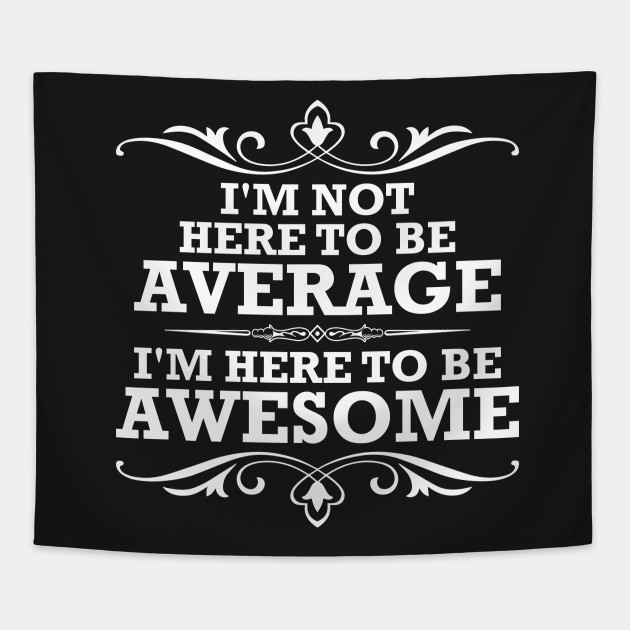
Update
Florian Hollenbach pointed out on twitter that Hanmer & Kalken (2013) discusses this very point! Very cool. Thanks, Florian!
References
Hanmer, M.J. and K.O. Kalkan (2013), "Behind the Curve: Clarifying the Best Approach to Calculating Predicted Probabilities and Marginal Effects from Limited Dependent Variable Models," American Journal of Political Science, 57(1), 263-277
Heckman, J. (1974), "Shadow Prices, Market Wages, and Labor Supply," Econometrica, 42(4), 679-694