Endogeneity with Measurement Error
Today I am going to take the opportunity to plug a short paper I wrote a few years ago. I remember a senior colleague telling me when I was an AP that as you age, you become much more concerned about your legacy. You write papers that you want to write, rather than worrying about tenure or other sources of external validation. The paper I am going plug falls squarely in this realm. I wrote it because I wanted to, regardless of what became of it.
As you can tell from my first few posts in this blog, I enjoy thinking about measurement error and endogeneity more generally. As both problems are very common in applied work, starting many years ago (yes, I am old) ...
I was increasingly coming across applied papers that confronted both issues. Specifically, papers that are interested in the causal effect of a covariate of interest on some outcome, but must confront the dual problems of said covariate being measured with error and being potentially endogenous (due to omitted unobserved variables) even in the absence of measurement error. In other words, even if measurement error did not exist, endogeneity would still be a problem and, most likely, instrument variable (IV) estimation is relied upon.
In such papers, often authors are still concerned about the "best" measure of the endogenous covariate, with "best" referring to a measure that reduces the measurement error in the covariate. To me, this raised a question. One solution to measurement error is IV. If a covariate is endogenous and IV is relied upon even if the "true" covariate was observed, does the the degree of measurement error matter?
Formally, what I have in mind is something like the following. The true model is
Y = Xb + cW* +e,
where Cov(W*,e) is non-zero so that W* is endogenous. However, W* is not observed, instead we observe W1 given by
W1 = W* + u1.
We can assume u1 is classical measurement error. Thus, the reliability ratio (RR) of W1 is
RR = Var(W*)/Var(W1)
which lies in the unit interval.
Assume you have access to a vector of strong and valid instruments, Z (and, if you believe that, I have some lovely swamp land to sell you).
The question is: Are my IV estimates "better" if I had a different mismeasured version of W*, W2, where
W2 = W* + u2
such that u2 is also classical measurement error and the RR of W2 is closer to one than the RR of W1?
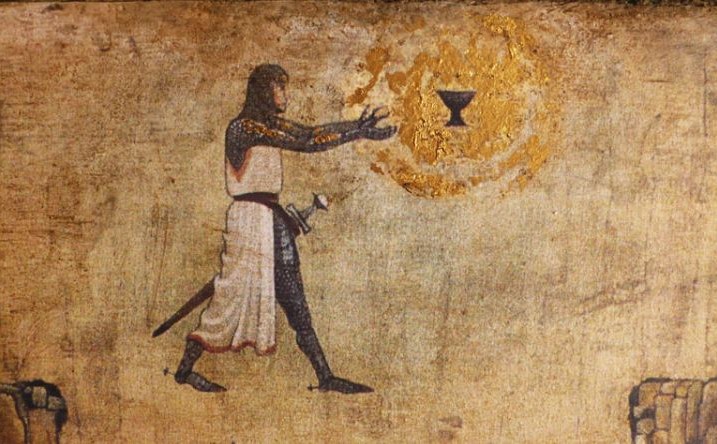
As you can tell from my first few posts in this blog, I enjoy thinking about measurement error and endogeneity more generally. As both problems are very common in applied work, starting many years ago (yes, I am old) ...
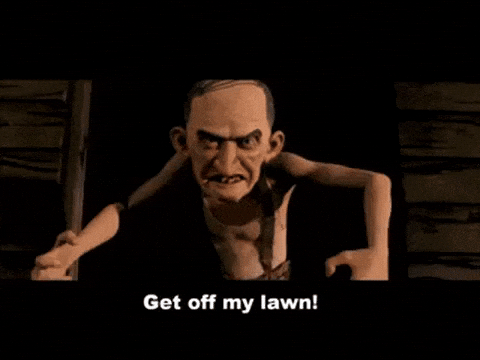
I was increasingly coming across applied papers that confronted both issues. Specifically, papers that are interested in the causal effect of a covariate of interest on some outcome, but must confront the dual problems of said covariate being measured with error and being potentially endogenous (due to omitted unobserved variables) even in the absence of measurement error. In other words, even if measurement error did not exist, endogeneity would still be a problem and, most likely, instrument variable (IV) estimation is relied upon.
In such papers, often authors are still concerned about the "best" measure of the endogenous covariate, with "best" referring to a measure that reduces the measurement error in the covariate. To me, this raised a question. One solution to measurement error is IV. If a covariate is endogenous and IV is relied upon even if the "true" covariate was observed, does the the degree of measurement error matter?
Formally, what I have in mind is something like the following. The true model is
Y = Xb + cW* +e,
where Cov(W*,e) is non-zero so that W* is endogenous. However, W* is not observed, instead we observe W1 given by
W1 = W* + u1.
We can assume u1 is classical measurement error. Thus, the reliability ratio (RR) of W1 is
RR = Var(W*)/Var(W1)
which lies in the unit interval.
Assume you have access to a vector of strong and valid instruments, Z (and, if you believe that, I have some lovely swamp land to sell you).

The question is: Are my IV estimates "better" if I had a different mismeasured version of W*, W2, where
W2 = W* + u2
such that u2 is also classical measurement error and the RR of W2 is closer to one than the RR of W1?
This question gnawed at me, in the recesses of my brain and my dreams at night, for a long time as I saw many papers where authors devoted presumably considerable research time trying to devise a W2 to replace W1 even though it did not eliminate the need for IV.

For example, when analyzing the effects of environmental regulations on economic outcomes, measures of regulation are likely mismeasured (since they are at best a proxy for true regulatory stringency) and correlated with unobserved determinants of relevant outcomes. However, much time and attention has been spent on devising "better" indices of environmental stringency.
In my paper ("Covariate Measurement and Endogeneity," Economics Letters, 2015, 136, 59-63), I show that the finite sample bias of IV (recall, IV is always biased in finite samples) is NOT monotonically decreasing in the RR of the endogenous covariate. In fact, in simulations, the bias of the IV estimator can be zero for certain RRs that are strictly less than one. Stated differently, it is a distinct possibility that by improving the RR of your proxy, the finite sample bias of IV may increase. Not a desirable outcome!
The conclusion to draw from the paper is important ... and it is NOT that obvious improvements in proxies for an endogenous covariate should be ignored. But, it does imply that devoting substantial researcher time to finding better proxies does not yield a big return (and the return may be negative). Instead, as the paper reminds us, the finite sample bias of the IV estimator is strongly influenced by the strength of the instruments AND the bias is monotonically decreasing in the strength of the instruments. So, the bottom line is that researchers are better off devoting their time to the elusive quest of finding stronger instruments.
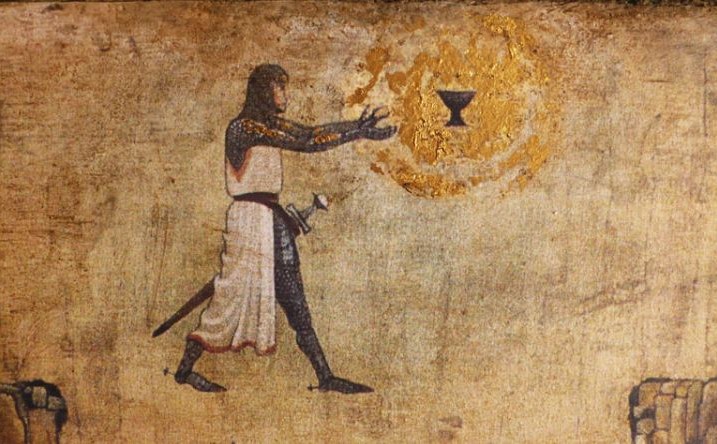
As long as I am plugging my paper, I also want to note here that two friends helped improve the paper tremendously. The first is Salvador Navarro who was visiting SMU at the time. The second is Le Wang, who is my former Ph.D. student and now endowed chair professor at U Oklahoma. It was so cool to learn from my former student.