Pick a Number
In case you were unaware, or I have neglected to mention it lately, measurement error exists and it is terrifying. Like the Yeti.
If you are researcher working with data, then let me tell you something: your data contain measurement error. Moreover, such error can wreak havoc with that wonderful empirical model you thought so long and hard to develop. When I first learned about measurement error in graduate school, I was so shocked that I almost did the unthinkable: become a theorist. Seriously.
Alas, my math skills would have prevented that even if I had wanted to go through with it.
So, back to measurement error. As I said, measurement error can be frightening because it can introduce bias into an otherwise correctly specified empirical model, even if the errors are completely random. If the errors are nonrandom, then all bets are off. While far from ideal, when a door shuts, a window opens. Or something like that.
Measurement error presents opportunities to reach beyond our comfort zone, learn new and interesting things, and better understand the world around us. To that end, this post is not about econometric solutions to measurement error, but rather about the detection of measurement error.
Most of what we know about measurement error comes from rare instances when we have one data source, known to be accurate, to validate a second data source. For example, workers participating in a survey might self-report whether or not they have employer-provided health insurance or if they are covered by a collective bargaining agreement. The researcher might then interview the respondents' employers and verify if the self-reported information is factual. Alternatively, individuals participating in a survey might self-report their education level. The researcher might then track down the self-reported credentials to verify their accuracy.
However, for most variables used by researchers, validation data are unavailable. What, then, is one to do? Well, fortunately, numbers are a curious thing. It also just so happens that erroneous numbers are an even curiouser thing.
See, numbers, when originating in the wild, tend to follow a pattern. Specifically, in many situations, the distribution of first significant digits of a variable is given by
where d is the first digit {1,2,...,9}. This is known as Benford's Law. Interestingly, Benford published this finding in 1938, several decades after Simon Newcomb published the same idea! Poor Simon. He's the Rodney Dangerfield of numbers.
In any event, it turns out that the distribution of first significant digits is often far from uniform; the probabilities are
Thus, lower digits are much more common that higher ones. Benford's Law does not always apply, but typically does when a variable spans several orders of magnitude. Examples include city or country populations around the world, income, pollution emissions, etc. Instances where the law does not apply include, perhaps most notably (because I wanted to use it for a paper!), proportions, which are bounded between 0 and 100.
To illustrate, I took the current distribution of country populations from here. The breakdown of first digits is
Pretty cool! And it is. But, the really cool part is what we can do with this new insight (if, indeed, this is new to you). Researchers (and governments) have put Benford's Law to good use. To detect measurement error!
If the first digits of a variable should follow Benford's Law, but the distribution of first digits in the data does not correspond to the distribution above, then Houston, as they say, we have a problem. The government uses this in forensic accounting to detect fraudulent record-keeping or tax evasion, and it has been proposed as a cost-effective way to detect fraudulent data in clinical trials. Benford's Law even made an appearance in an episode during Season 2 of ...
But, researchers make use of Benford's Law as well. And, now, you can too! It has even been extended to known distributions of second and higher digits, or combinations of leading digits.
I was reminded of this at our departmental brown bag presentation. The paper began by noting the well-known difficulty in measuring bilateral trade flows. For instance, say we wish to know how much stuff China exports to the US. Well, we have two measures of this in the data; Chinese data on exports to the US and US data on imports from China. The two are not the same even after adjustments for things such as trade costs.
To formally assess the intentional misreporting that may be occurring, the author examined the distribution of the first digits in the data on trade flows at the sectoral level. For many sectors, the distribution was not close. This allowed the author to use Benford's Law to not only identify the likely presence of measurement error, but also identify specific sectors that appear to be misreporting. Then, the author examines whether these particular sectors have more incentive to misreport. Like I said, pretty cool!
There are plenty of other examples of putting Benford's Law to good use as well. Previously, Benford's Law has been used by researchers to detect anomalies in pollution data. For example, the well-used Toxic Release Inventory (TRI) relies on self-reported emissions by firms and thus may be suspect (you think?). de Marchi & Hamilton (2006) use Benford's Law to show that TRI lead emissions likely contain significant misreporting, unlike data on lead emissions from EPA monitoring stations.
Way too many 5s! Kinda looks like firms might telling the government how they really feel!
Other recent examples in the empirical trade literature include Cerioli et al. (2019) and Demir & Javorcik (2018). Both examine customs data and the evasion of border taxes. The former also develops new statistical tests that may have wide applicability.
The data do not span orders of magnitude across countries, but it still conforms reasonably well, I think. So, then I downloaded current data on cases across US counties from here. Less variation perhaps, but larger sample. The results
Really close, huh?!
Numbers are indeed a curious thing. So, next time someone asks you to "pick a number," don't just pick any number.
Judge, G. and L. Schechter (2009), "Detecting Problems in Survey Data Using Benford's Law," Journal of Human Resources, 44(1), 1-24
Kaiser, M. (2019), "Benford's Law as an Indicator of Survey Reliability--Can We Trust Our Data?" Journal Economic Surveys, 33(5), 1602-1618
Koch, C. and K. Okamura (2020), "Benford’s Law and COVID-19 Reporting," Economics Letters, 196
Michalski, T. and G. Stoltz (2013), "Do Countries Falsify Economic Data Strategically? Some Evidence That They Might," Review of Economics and Statistics, 95(2), 591-616
Schündeln, M. (2018), "Multiple Visits and Data Quality in Household Surveys," Oxford Bulletin of Economics and Statistics, 80(2), 380-405
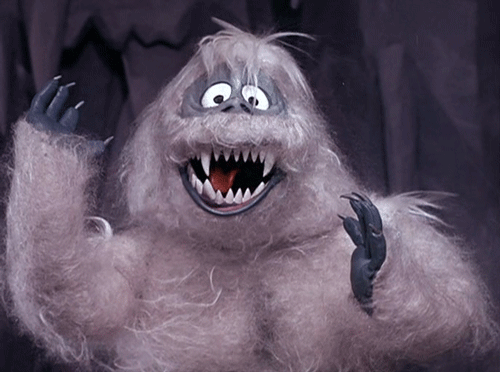
If you are researcher working with data, then let me tell you something: your data contain measurement error. Moreover, such error can wreak havoc with that wonderful empirical model you thought so long and hard to develop. When I first learned about measurement error in graduate school, I was so shocked that I almost did the unthinkable: become a theorist. Seriously.
Alas, my math skills would have prevented that even if I had wanted to go through with it.
So, back to measurement error. As I said, measurement error can be frightening because it can introduce bias into an otherwise correctly specified empirical model, even if the errors are completely random. If the errors are nonrandom, then all bets are off. While far from ideal, when a door shuts, a window opens. Or something like that.
Measurement error presents opportunities to reach beyond our comfort zone, learn new and interesting things, and better understand the world around us. To that end, this post is not about econometric solutions to measurement error, but rather about the detection of measurement error.
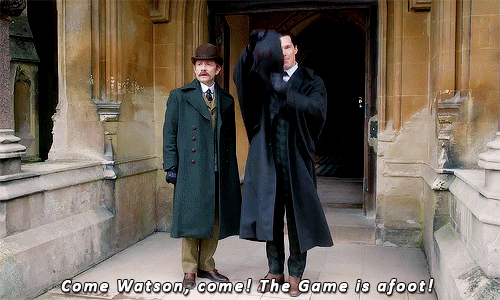
Most of what we know about measurement error comes from rare instances when we have one data source, known to be accurate, to validate a second data source. For example, workers participating in a survey might self-report whether or not they have employer-provided health insurance or if they are covered by a collective bargaining agreement. The researcher might then interview the respondents' employers and verify if the self-reported information is factual. Alternatively, individuals participating in a survey might self-report their education level. The researcher might then track down the self-reported credentials to verify their accuracy.
However, for most variables used by researchers, validation data are unavailable. What, then, is one to do? Well, fortunately, numbers are a curious thing. It also just so happens that erroneous numbers are an even curiouser thing.
See, numbers, when originating in the wild, tend to follow a pattern. Specifically, in many situations, the distribution of first significant digits of a variable is given by
where d is the first digit {1,2,...,9}. This is known as Benford's Law. Interestingly, Benford published this finding in 1938, several decades after Simon Newcomb published the same idea! Poor Simon. He's the Rodney Dangerfield of numbers.
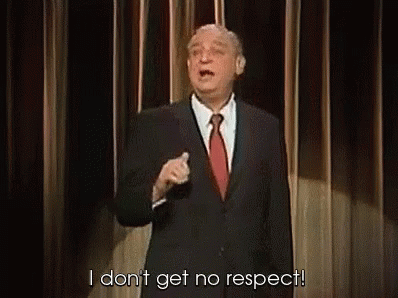
In any event, it turns out that the distribution of first significant digits is often far from uniform; the probabilities are
Thus, lower digits are much more common that higher ones. Benford's Law does not always apply, but typically does when a variable spans several orders of magnitude. Examples include city or country populations around the world, income, pollution emissions, etc. Instances where the law does not apply include, perhaps most notably (because I wanted to use it for a paper!), proportions, which are bounded between 0 and 100.
To illustrate, I took the current distribution of country populations from here. The breakdown of first digits is
Pretty cool! And it is. But, the really cool part is what we can do with this new insight (if, indeed, this is new to you). Researchers (and governments) have put Benford's Law to good use. To detect measurement error!

If the first digits of a variable should follow Benford's Law, but the distribution of first digits in the data does not correspond to the distribution above, then Houston, as they say, we have a problem. The government uses this in forensic accounting to detect fraudulent record-keeping or tax evasion, and it has been proposed as a cost-effective way to detect fraudulent data in clinical trials. Benford's Law even made an appearance in an episode during Season 2 of ...
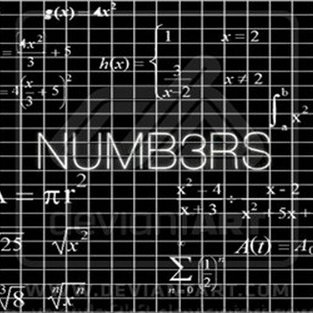
But, researchers make use of Benford's Law as well. And, now, you can too! It has even been extended to known distributions of second and higher digits, or combinations of leading digits.
I was reminded of this at our departmental brown bag presentation. The paper began by noting the well-known difficulty in measuring bilateral trade flows. For instance, say we wish to know how much stuff China exports to the US. Well, we have two measures of this in the data; Chinese data on exports to the US and US data on imports from China. The two are not the same even after adjustments for things such as trade costs.
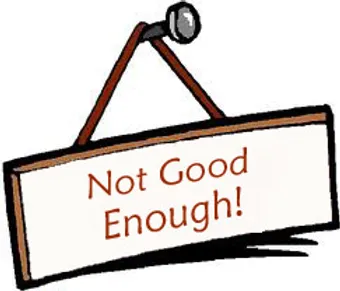
To formally assess the intentional misreporting that may be occurring, the author examined the distribution of the first digits in the data on trade flows at the sectoral level. For many sectors, the distribution was not close. This allowed the author to use Benford's Law to not only identify the likely presence of measurement error, but also identify specific sectors that appear to be misreporting. Then, the author examines whether these particular sectors have more incentive to misreport. Like I said, pretty cool!
There are plenty of other examples of putting Benford's Law to good use as well. Previously, Benford's Law has been used by researchers to detect anomalies in pollution data. For example, the well-used Toxic Release Inventory (TRI) relies on self-reported emissions by firms and thus may be suspect (you think?). de Marchi & Hamilton (2006) use Benford's Law to show that TRI lead emissions likely contain significant misreporting, unlike data on lead emissions from EPA monitoring stations.
Way too many 5s! Kinda looks like firms might telling the government how they really feel!
Other recent examples in the empirical trade literature include Cerioli et al. (2019) and Demir & Javorcik (2018). Both examine customs data and the evasion of border taxes. The former also develops new statistical tests that may have wide applicability.
Non-trade examples include Hickman & Rice (2010) who analyze crime report data in the US, Michalski & Stoltz (2013) who examine misreporting of country-level aggregate data along with incentives to misreport, and Schündeln (2018) who analyzes longitudinal surveys of consumption behavior. Schündeln (2018) uses Benford's Law to document a deterioration in self-reported consumption data when respondents are interviewed multiple times. Finally, Judge & Schechter (2009) and Kaiser (2019) offer two excellent overviews of both the use of Benford's Law as well as how one may formally test for divergences between distributions.
The critical takeaway here is that Benford's Law offers more than just an opportunity to know if your data are likely to contain significant measurement error. It offers an opportunity to ask new research questions. Specifically, why are some data misreported and not others? What are the incentives to misreport and are agents responding to those incentives? Fascinating.
You know what else is fascinating? COVID-19 data. Clearly, we are all worried about under-reporting in the data due to a lack of testing. Or, worse, intentional cover-ups by governments. Out of curiosity, I downloaded current country-level data on reported cases of COVID-19 from here. I had no idea what to expect, but here it is
The data do not span orders of magnitude across countries, but it still conforms reasonably well, I think. So, then I downloaded current data on cases across US counties from here. Less variation perhaps, but larger sample. The results
Really close, huh?!
Numbers are indeed a curious thing. So, next time someone asks you to "pick a number," don't just pick any number.
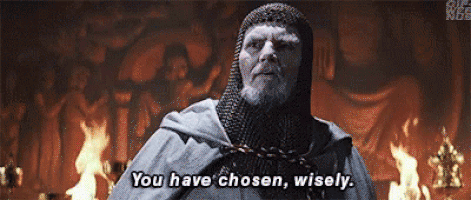
UPDATE (9.16.20)
Koch & Okamura (2020) use Benford's Law to test COVID-19 data from China.
References
Cerioli, A. L. Barabesi, A. Cerasa, M. Menegatti, and D. Perrotta (2019), "Newcomb–Benford Law and the Detection of Frauds in International Trade," PNAS, 116(1), 106-115
de Marchi, S. and J.T. Hamilton (2006), "Assessing the Accuracy of Self-Reported Data: An Evaluation of the Toxics Release Inventory," Journal of Risk & Uncertainty, 32, 57-76
Hickman, M.J. and S.K. Rice (2010), "Digital Analysis of Crime Statistics: Does Crime Conform to Benford’s Law?" Journal of Quantitative Criminology, 26, 333-349
Cerioli, A. L. Barabesi, A. Cerasa, M. Menegatti, and D. Perrotta (2019), "Newcomb–Benford Law and the Detection of Frauds in International Trade," PNAS, 116(1), 106-115
de Marchi, S. and J.T. Hamilton (2006), "Assessing the Accuracy of Self-Reported Data: An Evaluation of the Toxics Release Inventory," Journal of Risk & Uncertainty, 32, 57-76
Demir, B. and B. Smarzynska Javorcik (2018), "Forensics, Elasticities and Benford's Law," CESifo Working Paper No. 7266
Hickman, M.J. and S.K. Rice (2010), "Digital Analysis of Crime Statistics: Does Crime Conform to Benford’s Law?" Journal of Quantitative Criminology, 26, 333-349
Judge, G. and L. Schechter (2009), "Detecting Problems in Survey Data Using Benford's Law," Journal of Human Resources, 44(1), 1-24
Kaiser, M. (2019), "Benford's Law as an Indicator of Survey Reliability--Can We Trust Our Data?" Journal Economic Surveys, 33(5), 1602-1618
Koch, C. and K. Okamura (2020), "Benford’s Law and COVID-19 Reporting," Economics Letters, 196
Michalski, T. and G. Stoltz (2013), "Do Countries Falsify Economic Data Strategically? Some Evidence That They Might," Review of Economics and Statistics, 95(2), 591-616
Schündeln, M. (2018), "Multiple Visits and Data Quality in Household Surveys," Oxford Bulletin of Economics and Statistics, 80(2), 380-405