Skinning the Cat
In these trying times, we take comfort where we can find it.
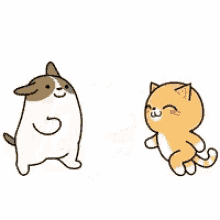
And, with everything else that's wrong in the world, one would think it cruel to take away a source of comfort to others. Nonetheless, this is exactly what happened this week on #EconTwitter, thanks to Paul Hünermund and Beyers Louw. In a just released working paper, Hünermund and Louw (2020) discuss a point that, while not new, certainly merits repeating for the benefit of researchers. Keele et al. (2020) discuss the same point as well. Reminding us of this point serves to effectively remove a source of comfort relied upon by researchers.

Not that I am calling Paul and Beyers cruel!
The point of this post is not simply to re-iterate the aforementioned papers. But, it is motivated by the above papers. And, to get there we must first talk about the above papers.
The setting is one in which the researcher wishes to estimate the causal effect of a treatment, D, on an outcome, Y, using observational data. A common way to proceed is to use Ordinary Least Squares (OLS) to estimate a model such as
Y = a + bD + cZ + e,
where Z is a vector of controls. Implicit in the estimating equation above is an underlying structure on the potential outcomes, Y(1) and Y(0), and treatment assignment. One might be willing to assume conditional (on observed variables) independence (CIA), along with particular functional forms for the potential outcomes, such that OLS will produce an unbiased estimate of b. However, because of the fundamental problem of causal inference, so-named in Holland (1986) and discussed in my previous post here, the CIA is not testable.
To overcome the lack of formal tests, researchers typically look for signs of the plausibility of CIA.

One such sign used by researchers, and the subject of the papers referenced above, is the OLS estimate of c. In particular, is the estimate of c "reasonable" and of the "correct" sign? The (valid!) conclusion in both papers is that this is silly.
As is well articulated in both papers, whether or not b has a causal interpretation is distinct from the question of whether or not c has a causal interpretation. And, if c does not have a causal interpretation, then the issue of its "reasonableness" is moo.
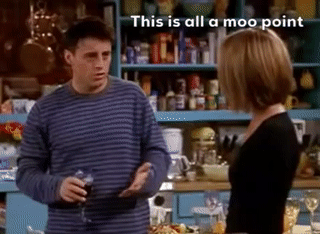
Hünermund and Louw (2020) start with a fairly simple DAG. Yes, I'm going to use DAGs.

But, I'm going to make it a bit more complex. So, let's start with the following DAG.
The model reflects the following:
- Y depends on D, Z1, Z2, and noise (ey)
- D depends on Z1 and noise (ed)
- Z1 depends on u and noise (e1)
- Z2 depends on u and noise (e2)
- Only Y, D, and Z1 are observed
With this structure, OLS estimates of the regression of Y on D are biased for the causal effect of D on Y. However, OLS estimates of the regression of Y on D and Z1 are unbiased for the causal effect of D on Y, but biased for the causal effect of Z1 on Y. Thus, the "reasonableness" of the coefficient on Z1 is an inappropriate sign for the "reasonableness" of the coefficient on D. Likewise, it is trivial to come up with a DAG where the OLS coefficient on D would be biased for the causal effect of D on Y, but unbiased for the causal effect of Z1 on Y. Again, the "reasonableness" of one coefficient in no way guarantees the "reasonableness" of another.
Let's turn to the regression model so we can use some econometric, non-DAG terminology. The model being estimated is
Y = a + b*D + c1*Z1 + error.
With the preceding structure, the OLS estimate of c1 does not represent the causal effect of Z1 on Y because Z1 is endogenous (i.e., Z1 is correlated with the regression error since Z2 is relegated to the error and Corr(Z1,Z2|D) is non-zero). Nonetheless, the OLS estimate of b does represent the causal effect of D on Y.
As I said, this is the point of the above papers. But, this is not the point of this post.
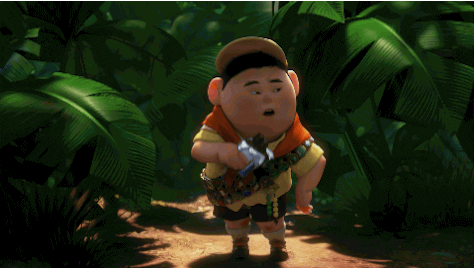
The point, as Paul and I have discussed on and off a few times, is that the DAG above really, really -- did I mention really -- bothers me. And not just because it is a DAG. Why then? Let me tell you.
In my econometrics classes, I preach to my students:
"Do not ignore the endogeneity of covariates just because they are not of interest"
I do this because typically if a covariate is endogenous, but this endogeneity is ignored, then not only is its coefficient biased, but also the coefficient of other covariates that are correlated with it. Well, that's not true in the above DAG! With the preceding structure, in the regression model
Y = a + b*D + c1*Z1 + error,
Z1 is falsely treated as exogenous, Z1 is correlated with D, but the OLS coefficient on D is unbiased nonetheless.
So, my explanation to my students is not true, at least not always. What, then, is going on? I am quite positive that the real econometricians reading this blog -- of which I am not (see here) -- have a precise explanation (see, e.g., Frölich (2008)). And that's great. But, that explanation may not be internalized by most applied researchers. It's likely to be met with glossy eyes and boredom. Hopefully, my dive into this topic will be met with at least less eye-rolling.
At the outset, I want to emphasize that the DAG in Hünermund and Louw (2020) bothers me tremendously not because Hünermund and Louw (2020) are wrong (certainly not!), and not because we ought not be very careful interpreting the coefficients on covariates other than the treatment, but because I worry it will give false comfort to researchers conditioning on endogenous covariates. In the DAG above, failing to condition on Z1 produces a biased estimate of the causal effect of D on Y. But, conditioning on an endogenous covariate produces an unbiased estimate of this effect.
For those well versed in DAG-speak, it is clear from the picture that conditioning on Z1 "works" because it blocks the backdoor path from D to Y. In econometrics-speak, it "works" because the partial correlation between D and Z2 (the regression error), given Z1, is zero despite the fact that the unconditional correlation is non-zero. And, this lack of partial correlation is also apparent in the DAG to those familiar with the rules of d-separation.
But, let's back up a second. The basic data-generating process (DGP) being depicted in the DAG above is one with the following attributes:
- Y depends on D, Z1, Z2, and noise
- D is correlated with Z1
- Z1 is correlated with Z2
- Only Y, D, and Z1 are observed
My contention is that (i) there are lots of ways one could draw a DAG that corresponds to this structure, (ii) the properties of OLS will differ markedly across them, and (iii) researchers would be hard-pressed to use institutional knowledge in a particular application to distinguish between them.
Exhibit 1.
In this DAG,
- D is correlated with Z1 through e1
- Z1 is correlated with Z2 through u
- Only Y, D, and Z1 are observed
The point of this case is to illustrate that the unbiasedness of OLS for the causal effect of D on Y depends on how D and Z1 are correlated. Is it a direct effect as in Hünermund and Louw (2020), or is it due to a common factor, e1? Can a researcher really make a case for which DAG represents the true DGP in a particular application?
The astute reader might mention that in Hünermund and Louw (2020), the unconditional correlation between D and Z2 is non-zero, whereas it is zero in Exhibit 1. Perhaps this is useful to know, but let's move on.
Exhibit 2.
In this DAG, the only difference from Exhibit 1 is that now Z2 also depends on e1. In this case, conditioning on Z1 also fails to produce an unbiased estimate of the causal effect of D on Y. But, in my simulations (at the end of this post), it does. Paul says this is due to identification via functional form and, as such, is not a general result which is why Daggity says it doesn't work. [Note: My simulation breaks down if I instead generate Z2 = 2u+e1+e2.] Econometrically, I see why it works with the functional forms I consider; the partial correlation between D and Z2 is again zero. But, in general, we have a situation where D is unconditionally correlated with both Z1 and Z2 and OLS is no longer unbiased for the causal effect of D on Y. Moreover, testing whether the partial correlation between D and Z2 is zero is not possible -- absent further information -- given that Z2 is unobserved.
Exhibit 3.
There is no new DAG here. Instead, in my simulations, I use Stata's -drawnorm- command to generate D, Z1, and Z2 from a trivariate normal distribution such that Corr(D,Z1) and Corr(Z1,Z2) are non-zero, while Corr(D,Z2) is zero. Stata's command generates variables from a multivariate normal distribution with fixed correlation matrix using a Cholesky decomposition of the desired variance-covariance matrix. Proceeding this way leads to data where the partial correlation between D and Z2 is non-zero despite the unconditional correlation being zero. Thus, neither conditioning on or omitting Z1 from the regression model will yield an unbiased estimate of the causal effect of D on Y.
Exhibit 4.
This is a DAG with a single covariate that is, waiiiittttt for it, measured with error. Z* is the true covariate, z is the mismeasured version, and u is the measurement error. In this case, again, neither conditioning on or omitting Z from the regression model will yield an unbiased estimate of the causal effect of D on Y.
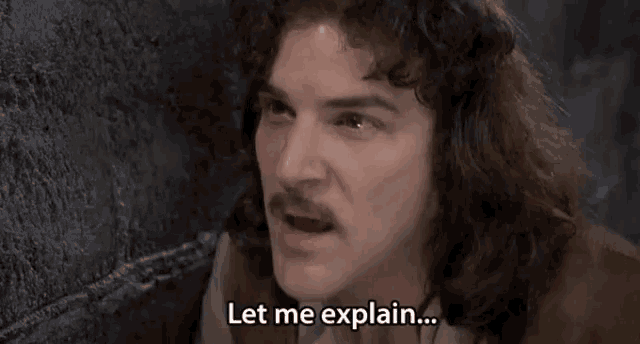
Let me effort to sum up. If you condition on an endogenous covariate, beware!
Clearly conditioning on an endogenous covariate will never produce an unbiased estimate of the causal effect of the covariate. Whether it helps you obtain an unbiased estimate of the causal effect of the treatment is highly dependent on very subtle differences in the DGP. Differences that I claim would be very hard to parse based on the usual institutional knowledge that a researcher possesses. Reminds me of a line I came across this week in the book I am currently reading, The Neruda Case
"But life is an iceberg, Cayetano. We can't see the most essential parts."
This week, I also had the idiom
"There is more than one way to skin a cat"
stuck in my head for some reason. It, too, applies here. There is more than one DAG that captures the critical features of the DGP I have focused on thanks to Hünermund and Louw (2020)
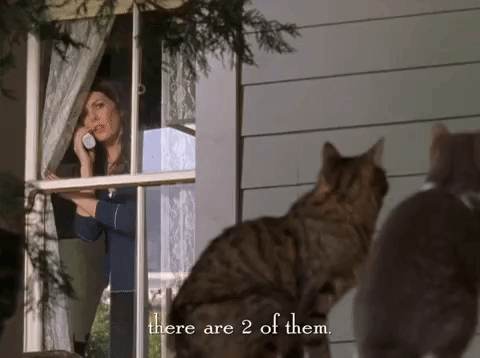
There are even more than two! And, when Z2 is unobserved, which DAG is correct matters tremendously and is very likely unknown. Fundamentally, I believe this is so because it is very hard for us to think about partial correlations. That is the crux.
In any regression model that involves D and stuff, what we need is that the partial correlation between D and the regression error is zero (i.e., d-seperation). Consequently, I could channel my inner Inigo Montoya and sum up both Hünermund and Louw (2020) and this post with simply this
"Only interpret coefficients on covariates whose partial correlation with the regression error is zero"
Something along these lines is what I envision a real econometrician would say. But, partial correlations are not intuitive. As a result, this summation is not of practical value to researchers, in my view. So, I will revert back to what I teach my students.
"Do not ignore the endogeneity of covariates just because they are not of interest"
Doing so should bring you no comfort. Just like DAGs for me.
H/t
Special thanks to Paul for humoring my attempt to use DAGs, discussing these issues on multiple occasions, and commenting on this post. Thanks also to David Drukker for discussing Stata's -drawnorm- command. If you don't know David, he was head of econometrics at Stata for more than a decade (perhaps two) and is now a professor in the economics department at Sam Houston State U.
References
Frölich, M. (2008), "Parametric and Nonparametric Regression in the Presence of Endogenous Control Variables," International Statistical Review, 76(2), 214-227
Holland, P. (1986), "Statistics and Causal Influence," Journal of the American Statistical Association, 81, 945-960
Hünermund, P. and B. Louw (2020), "On the Nuisance of Control Variables in Regression Analysis," unpublished manuscript. https://arxiv.org/abs/2005.10314.
Keele, L., R.T. Stevenson, and F. Elwert (2020), "The Causal Interpretation of Estimated Associations in Regression Models," Political Science Research and Methods, 8, 1-13
Simulation in Stata
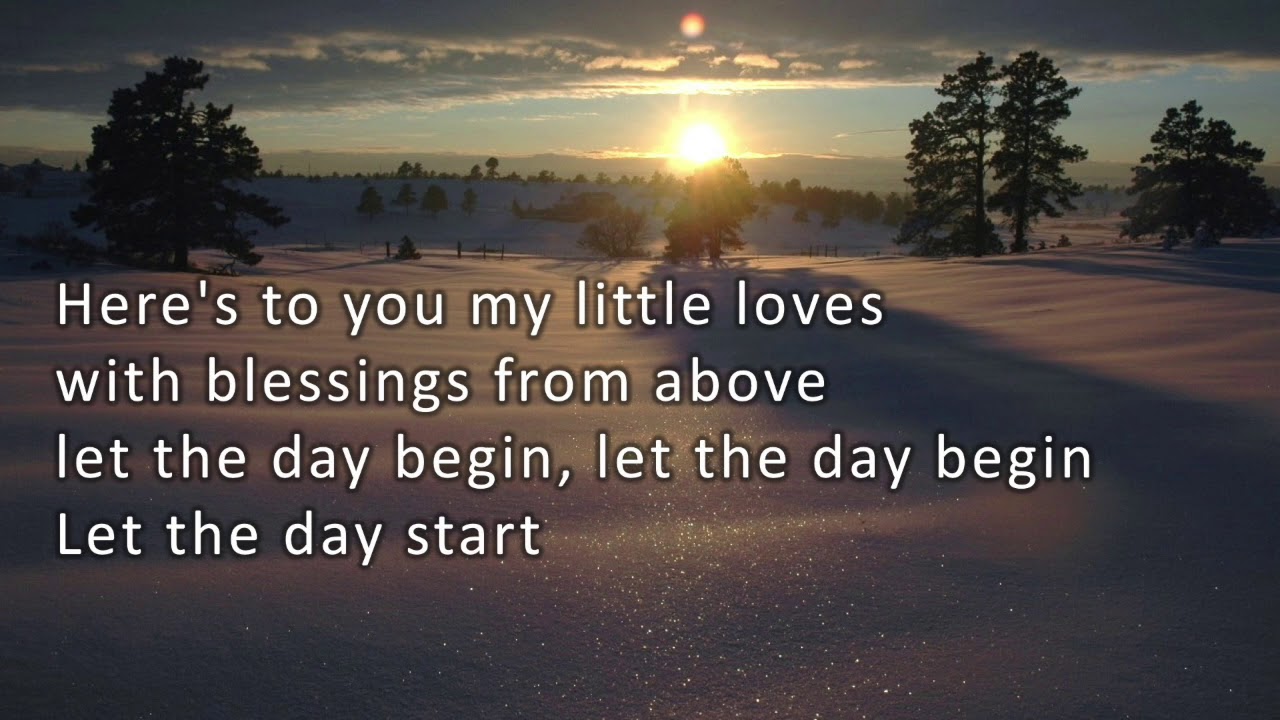
Do File:
qui {
clear all
set obs 100000
g u=rnormal()
g e1=rnormal()
g e2=rnormal()
g z1=u+e1
g z2=u+e2
g ed=rnormal()
g D=z1+ed
g y=D+z1+z2+rnormal()
noi di "EXHIBIT 0. Hünermund and Louw (2020): Corr(D,z2)ne0, Corr(z1,z2)ne0, Corr(D,z2|z1)=0"
reg D z1
predict Dres, res
noi corr D Dres z2
reg y D z1
eststo m0
reg y D
eststo m0a
noi di "EXHIBIT I. Corr(D,z2)=0, Corr(z1,z2)ne0, Corr(D,z2|z1)ne0"
drop D y Dres
g D=e1+ed
g y=D+z1+z2+rnormal()
reg D z1
predict Dres, res
noi corr D Dres z1 z2
reg y D z1
eststo m1
reg y D
eststo m1a
noi di "EXHIBIT II. Corr(D,z2)ne0, Corr(z1,z2)ne0, Corr(D,z2|z1)=0"
drop y Dres z2
g z2=u+e1+e2
g y=D+z1+z2+rnormal()
reg D z1
predict Dres, res
noi corr D Dres z1 z2
reg y D z1
eststo m2
reg y D
eststo m2a
noi di "EXHIBIT III. Corr(D,z2)=0, Corr(z1,z2)ne0, Corr(D,z2|z1)ne0"
drop D z1 z2 y Dres
mat C = (1,.5,0 \ .5,1,-.5 \ 0,-.5,1)
mat list C
drawnorm D z1 z2, corr(C)
g y=D+z1+z2+rnormal()
reg D z1
predict Dres, res
noi corr D Dres z1 z2
reg y D z1
eststo m3
reg y D
eststo m3a
noi estout m0 m1 m2 m3, cells(b(star fmt(3)) se(nopar fmt(3))) style(fixed) stats(N R2, fmt(%9.0g 3)) starlevels(* 0.10 ** 0.05 *** 0.01) legend
noi estout m0a m1a m2a m3a, cells(b(star fmt(3)) se(nopar fmt(3))) style(fixed) stats(N R2, fmt(%9.0g 3)) starlevels(* 0.10 ** 0.05 *** 0.01) legend
clear all
set obs 100000
noi di "EXHIBIT IV. Corr(D,u)=0, Corr(z,zstar)ne0, Corr(D,u|z)ne0"
g zstar=rnormal()
g u=rnormal()
g z=zstar+u
g ed=rnormal()
g D=zstar+ed
g y=D+zstar+rnormal()
reg D z
predict Dres, res
noi corr D Dres z u
reg y D z
eststo m4
reg y D
eststo m4a
noi estout m4 m4a, cells(b(star fmt(3)) se(nopar fmt(3))) style(fixed) stats(N R2, fmt(%9.0g 3)) starlevels(* 0.10 ** 0.05 *** 0.01) legend
}
Output:
EXHIBIT 0. Hünermund and Louw (2020): Corr(D,z2)ne0, Corr(z1,z2)ne0, Corr(D,z2|z1)=0
(obs=100,000)
| D Dres z2
-------------+---------------------------
D | 1.0000
Dres | 0.5778 1.0000
z2 | 0.4086 0.0015 1.0000
EXHIBIT I. Corr(D,z2)=0, Corr(z1,z2)ne0, Corr(D,z2|z1)ne0
(obs=100,000)
| D Dres z1 z2
-------------+------------------------------------
D | 1.0000
Dres | 0.8668 1.0000
z1 | 0.4987 -0.0000 1.0000
z2 | -0.0017 -0.2894 0.4996 1.0000
EXHIBIT II. Corr(D,z2)ne0, Corr(z1,z2)ne0, Corr(D,z2|z1)=0
(obs=100,000)
| D Dres z1 z2
-------------+------------------------------------
D | 1.0000
Dres | 0.8668 1.0000
z1 | 0.4987 -0.0000 1.0000
z2 | 0.4077 0.0007 0.8163 1.0000
EXHIBIT III. Corr(D,z2)=0, Corr(z1,z2)ne0, Corr(D,z2|z1)ne0
(obs=100,000)
| D Dres z1 z2
-------------+------------------------------------
D | 1.0000
Dres | 0.8674 1.0000
z1 | 0.4975 -0.0000 1.0000
z2 | 0.0057 0.2915 -0.4968 1.0000
m0 m1 m2 m3
b/se b/se b/se b/se
D 1.003*** 0.666*** 0.997*** 1.332***
0.005 0.004 0.004 0.005
z1 1.496*** 1.665*** 1.999*** 0.336***
0.006 0.004 0.004 0.005
_cons -0.003 0.003 -0.002 0.001
0.005 0.005 0.004 0.004
N 100000 100000 100000 100000
R2
* p<0.10, ** p<0.05, *** p<0.01
m0a m1a m2a m3a
b/se b/se b/se b/se
D 2.002*** 1.498*** 1.996*** 1.499***
0.004 0.006 0.006 0.004
_cons -0.006 0.006 0.001 0.000
0.006 0.008 0.009 0.004
N 100000 100000 100000 100000
R2
* p<0.10, ** p<0.05, *** p<0.01
EXHIBIT IV. Corr(D,u)=0, Corr(z,zstar)ne0, Corr(D,u|z)ne0
(obs=100,000)
| D Dres z u
-------------+------------------------------------
D | 1.0000
Dres | 0.8684 1.0000
z | 0.4959 0.0000 1.0000
u | -0.0048 -0.4084 0.7054 1.0000
m4 m4a
b/se b/se
D 1.333*** 1.499***
0.003 0.003
z 0.335***
0.003
_cons -0.003 -0.002
0.004 0.004
N 100000 100000
R2
* p<0.10, ** p<0.05, *** p<0.01