Chicken or Egg?
It's a question nearly as old as time itself, dating back at least to Aristotle.
"Which came first, the chicken or the egg?"
Individuals representing different disciplines have arrived at different answers, or no answer at all.
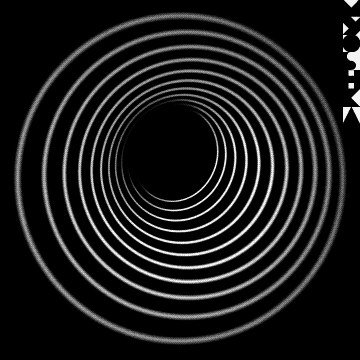




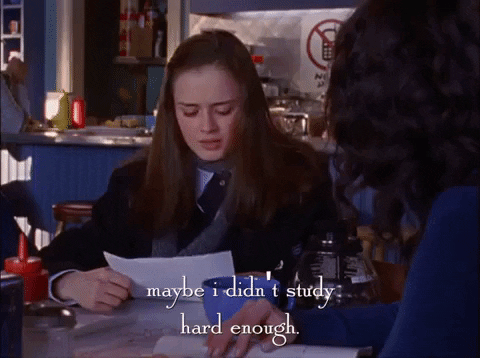
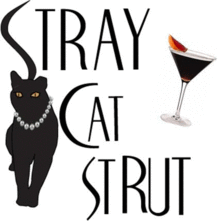
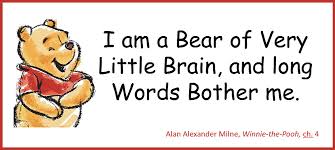
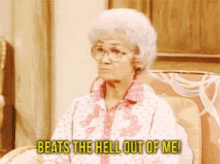
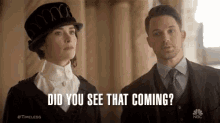

I've been thinking about this ancient question lately, and not for the reasons you might think. It would be easy to link this question to questions of causation that many readers of this blog grapple in their empirical research. Does the egg cause the chicken to exist, or does the chicken cause the egg to exist. I'm sure if Paul is reading this -- you know which Paul -- his mind is immediately going to what the DAG looks like and, I imagine, concluding that it is not acyclic.
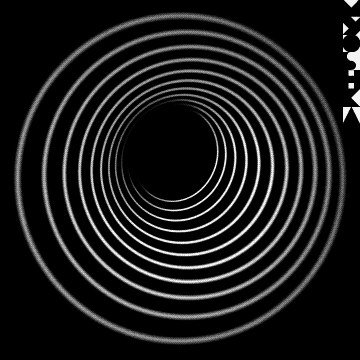
Anyway, I digress. This post is not about causation per se. This is about an issue in empirical work that rears its "ugly" head far too often, under different guises, different motivations, different descriptions. But, nonetheless, fundamentally represent the same underlying issue.

The issue to which I am referring can be cast as the following question:
"Which comes first, the model or the estimator?"
Unlike the question of the chick and the egg, this question has a correct answer, in my view and I think in the view of most. I know for sure that two econometricians I respect highly -- Pedro Sant'Anna and Sal Navarro -- believe this question has a correct answer.

The answer is that the model must come first. Estimation, including both choice of estimator and data considerations, must come second.
Let's dive into this because, as I said above, I view the question of model versus estimator as referring to many different situations. To start, let's define things so we are on the same page. I view the model as anything from the most simple statement to the most complex structural model that one might envision. The bottom line is that the model begins with one's assumptions concerning the true data-generating process (DGP). This DGP is then used to define the population parameter(s) that we wish to learn something about, their interpretation of which becomes clear in the context of the DGP.
This DGP could be as straightforward as parametric, reduced form specification, or it could be some convoluted -- I mean, brilliant! -- structural model.

For example, when using the potential outcomes framework, the DGP begins with functional forms for the potential outcomes. This then allows one to define the agent-specific treatment effect as the difference in potential outcomes for a given agent, and then define the parameter(s) of interest, such as the average treatment effect (ATE) in the population. The population object(s) that we are trying to learn something about are also referred to as the estimand(s).
Once we know what we wish to learn something about, we can then -- and only then -- turn to the issue of how to learn something about it from the data at hand. Here, the researcher proposes a particular estimator and hopefully discusses the properties of said estimator given the assumed DGP. If the estimator yields a point estimate, we can discuss whether it is unbiased (a finite sample property) and/or consistent (an asymptotic property), as well as it finite and asymptotic variance. If the estimator yields a set of points, we can discuss the size of this set and its sharpness.
This probably all sounds completely innocent and straightforward. We all proceed in this order. True. Except when we do not.
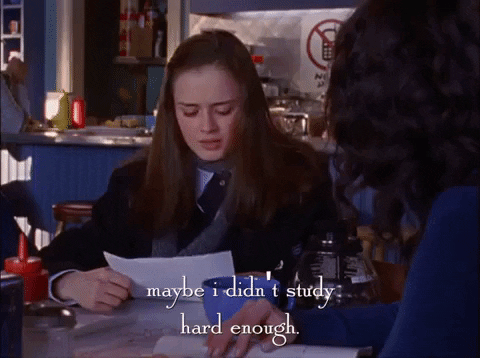
So, when do we deviate from this strict order?
The most common situation is where researchers know the estimator they intend to use and thus start there, skipping over thinking about the true, underlying DGP. Proceeding in this way can lead to issues in terms of interpreting the meaning and properties of one's estimate.
As an example, see this recent Twitter thread by Pedro related to Słoczyński (2020). Słoczyński shows that if one starts with the estimator, in this case a multivariate OLS regression with a treatment dummy, the OLS estimate of the coefficient on the treatment dummy does not estimate what the researcher may think it does if the treatment effect is agent-specific. The idea is similar in spirit to the failure of difference-in-differences (DID) to estimate a meaningful parameter in the presence of heterogeneous treatment effects and staggered treatment timing. In both situations, by deciding on the estimator before considering the underlying model, one is (usually) led astray.
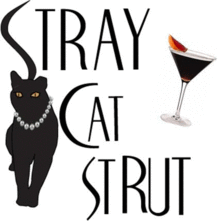
Another example is one that I encountered about a decade ago when working on an empirical trade paper. I was recently reminded of it while discussing a more recent trade paper with Mary Lovely. The problem that frustrated both her and I is the following. With access to panel data, some trade researchers were jumping immediately to the estimator by starting with the equation to be estimated by pooled OLS, where the dependent variable is written in terms of changes (e.g., first-differences) and the covariates may be some combination of changes and/or levels. This approach really bothers us because my simple brain -- Mary's works much better -- has a difficult time envisioning the model the researcher has in mind, let alone evaluating that model, when it begins by specifying outcomes in changes.
Instead, I want to first know the model envisioned by the researcher in order to evaluate its reasonableness as well as understand what the estimator being used is estimating. For that, I want to think about how the relevant outcome is determined in levels, which is much more intuitive and easier to evaluate. Starting with an estimating equation in levels may reveal different problems than those documented in Słoczyński (2020), but the problems start from the same source: a failure to carefully think through the model before jumping to an estimating equation and estimator.
A third example may be even more common than those already discussed. It is something I saw in my the first referee report I ever did as a graduate student, and it is something I see many times over the years from my graduate students. I'm sure I have been guilty of it as well.
The problem is letting the estimator you intend to use -- which, importantly, also encompasses the data you intend to use -- dictate the model you specify. Often, researchers know from the outset that key variables they will need are unobserved. Confronted with such an issue, researchers typically resort to writing down the estimator they can implement, given the available data. But, how can the resulting estimate be interpreted and its properties evaluated? They cannot.
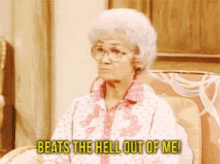
Instead, one should always start by thinking about the true, underlying DGP without consideration of estimation and data limitations. From this, one can discuss the estimator. Proceeding in this order allows one to evaluate the properties of the estimator in light of the assumed DGP and the fallibility of the data; alternatively, it allows one to articulate in a transparent manner what needs to be assumed for the estimator to be interpreted in a particular manner. Otherwise, we are just left to guess work.
My discussion here may be a bit too abstract and incoherent to properly convey my thoughts. So, let me illustrate. Measurement error actually provides an easy example.
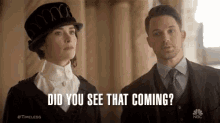
A researcher might be interested in the effect of x* on y. However, they may know that x* is not available in the data; instead, there is something related, a proxy x. So, the researcher proceeds to start the methods section of the paper writing down a regression equation for y on x.
Proceeding in this manner puts the estimator before the model. The researcher is jumping to the estimator -- a regression of y on x -- without first specifying the model. Without a complete model, it is not possible to understand or evaluate what the meaning and the properties of the OLS estimate. Instead, the researcher should start by specifying the true, underlying DGP. This might be as simple as assuming that
y = a + bx* + e.
Then, realizing that this model cannot be estimated due to a lack of data on x*, the researcher might opt to augment the DGP to include assumptions about how x is generated in the population as well. Given this complete model, the researcher can then figure out what it means to regress y on x, specifically what is the interpretation and properties of the estimator.

Ardent defenders of structural economics, such as Sal, are probably thinking, "What do you think we have been saying all along?" Or, as he actually wrote (playfully) on Twitter this week in a conversation about structural models,
"It is science, but I am sure it sounds like magic to you infidels."
While structuralists are correct, you do not need to be, nor am I advocating, we all become structural econometricians in order to ensure that the model comes before the estimator. Instead, we simply need to be better about separating the model from the estimation. In my view, one must always start with what you believe the true DGP to be and convince the reader of this. Then, and only then, manipulate the DGP, possibly by expanding upon it, to get to a model that is estimable. Finally, interpret the parameters of your estimable model and evaluate the properties of your estimation technique.
To borrow another cliche, don't do this ...
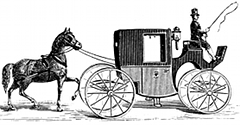
Instead, do this ...

References
Słoczyński, T. (2020), "Interpreting OLS Estimands When Treatment Effects Are Heterogeneous: Smaller Groups Get Larger Weights," Review of Economics & Statistics, conditionally accepted