Frame Job
The world is grappling with extremely important issues, both inside academia and economics in particular and outside in the real world. I was struggling with whether to write a post this week and was leaning towards not. Then I came across an interesting Twitter thread by Ioana Marinescu which linked to an earlier thread by Mar Reguant. I became inspired to write a post for those with the mental bandwidth, or those needing a brief (and partial) respite.


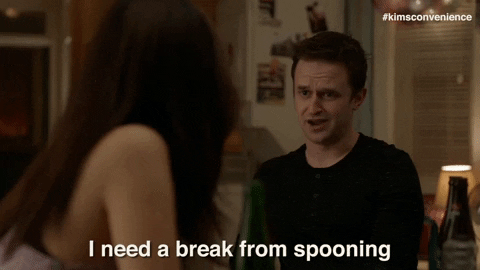
The subject of the threads, particularly the one by Mar, is testing for the presence of racism. To understand the issue, let's back up and briefly review what it means (in economics) to conduct a statistical test.
Before I do, I would like to acknowledge that I have seen several scholars on Twitter espouse the position that researchers are devoting too much time testing for the presence of racism. People of Color know racism exists. Thus, efforts should instead be directed to finding solutions. I defer to those more knowledgeable on this point. The objective of this post is simply to discuss statistical testing as it relates to testing for racism or any other phenomenon if one wishes to do so.
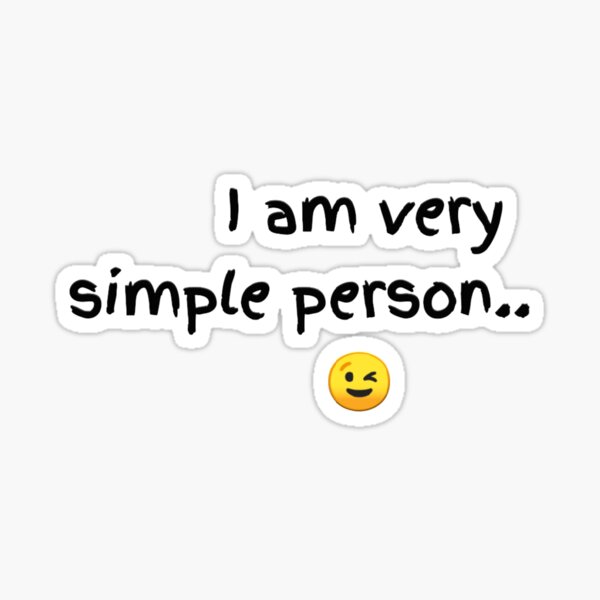
Now, let's return to the problem at hand. In economics, empirical testing is performed nearly universally within the null hypothesis significance testing (NHST) framework. With NHST, the researcher specifies a null and alternative hypothesis. The null hypothesis is tested (against the alternative) by first deriving a test statistic. A test statistic is a random variable that has a known distribution under the null (i.e, in the world in which the null hypothesis is true) and any other distribution under the alternative (i.e., in any world in which the alternative hypothesis is true).
After computing the value of this test statistic, we can find the p-value, a frightening creature if there ever was one (e.g., Kuffner & Walker 2019).

The p-value is the probability of drawing a random sample the same size as your data set in the world in which the null hypothesis is true and obtaining a value of the test statistic as extreme or more extreme than the value of the test statistic realized in your data set. That's a mouthful!
Finally, we (tend to) draw a binary conclusion if the p-value is greater than or less 0.05 (e.g., Kennedy-Shaffer 2019).

While controversies surround the misinterpretation of p-values as well as the choice to draw binary decisions using (arbitrary) thresholds such as 0.05, most empirical researchers are aware of all this. What researchers tend to think less about is the framing of the null and alternative hypotheses in the first place. But, this is crucial because NHST entails a bias toward the null hypothesis.
What does this mean? It means that with NHST a comparison is made between the realized p-value and arbitrary thresholds such as 0.05 to draw binary conclusions regarding the validity of the null and alternative hypotheses. I use the vague term "validity" here intentionally. If one wishes to use a significance level of 0.05, then a p-value exceeding this threshold implies that one fails to reject the null hypothesis. It is critical that we use we the phrase "fail to reject" and not "accept." Acceptance implies that we believe the null hypothesis to be true. But, a p-value exceeding 0.05 does not mean that the null is necessarily true. Instead, it means that the data do not provide sufficient evidence to disprove it.

On the other hand, if the p-value is less than 0.05, then the data provide sufficiently strong evidence disputing the truthfulness of the null hypothesis and one can say that it is rejected.
Stepping back, this means that despite the null hypothesis never being accepted, we fail to reject it only if the evidence is overwhelming against it. This is the source of the bias toward the null hypothesis with NHST. The null hypothesis is only rejected if the data provide overwhelming evidence against it.
The consequence of this bias is that researchers need to think carefully about how they are framing their tests when they choose which hypothesis to assign as the null and which to assign as the alternative. The result of your test is not invariant to this choice!

This brings us back to the Twitter feeds that I mentioned at the outset. Ioana and Mar both make the point that when researchers do set forth to test for the presence of racism, the null hypothesis is (always?) "no racism" and the alternative hypothesis is that "racism exists." This choice implies that the researcher will only reject the null hypothesis of no racism if the data contain overwhelming evidence of racism. As Mar states in her thread, "Let that sink in."
What then is the solution? One possibility is to look at the literature on non-nested model comparisons. When comparing two models, we say that they are non-nested if neither model is a special case (i.e., restricted version) of the other. If we wish to test one model versus another model, and the two models are non-nested, we want to treat the models symmetrically. We do not want to use a testing procedure that is biased towards one of the two models when we have no reason to play favorites.
To accomplish this, testing procedures -- such as the J-test or the non-nested F-test -- conduct two tests, allowing each model to have a turn as the null hypothesis. Then, one model is deemed to be rejected (in favor of the other) if the null hypothesis is rejected when this model is the null and the null hypothesis is not rejected when this model is the alternative. If the null hypothesis is rejected in both cases or not rejected in both cases, then the results are ambiguous as the data are insufficient to distinguish between the two models.
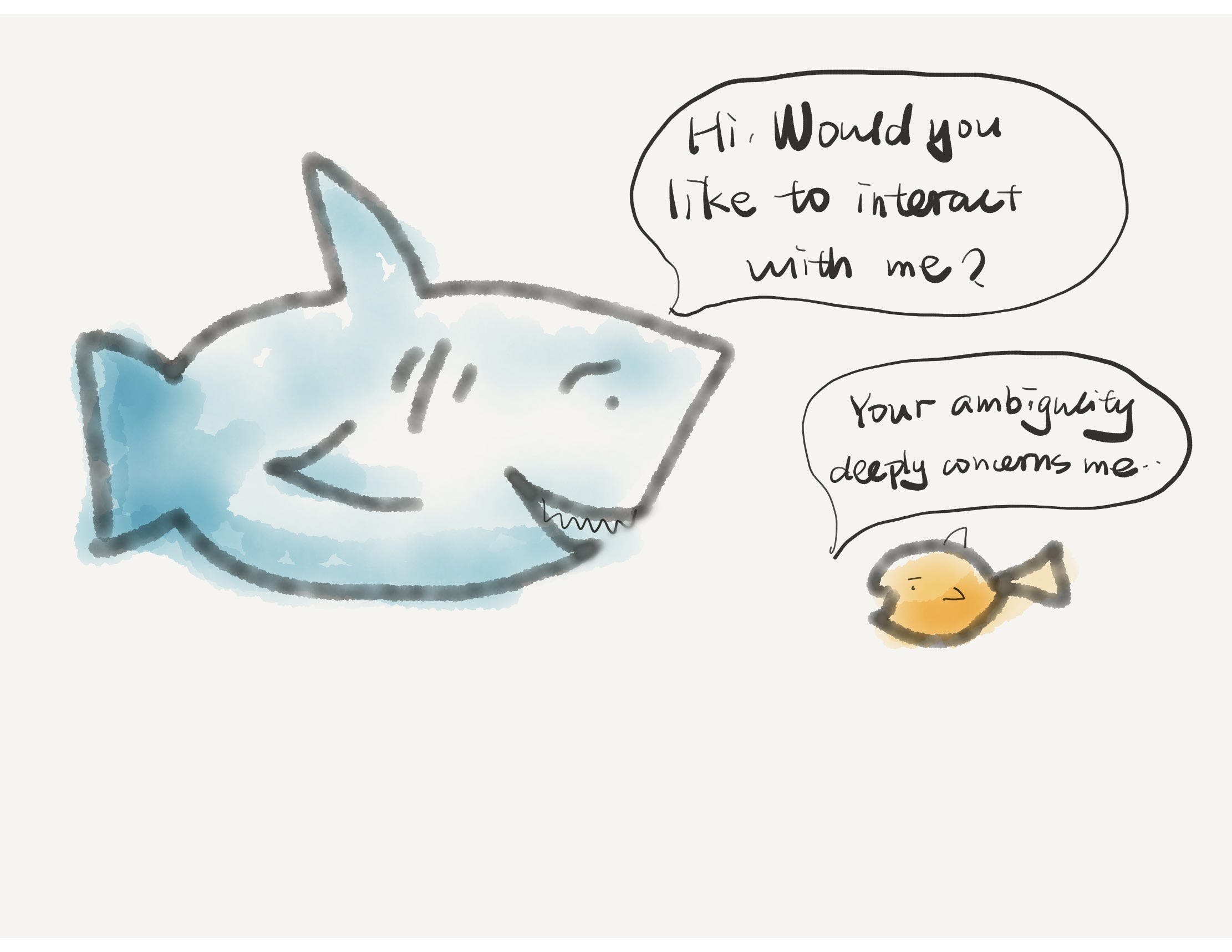
When testing for the presence of racism, something similar could be done. If we do not wish to bias the testing procedure in a particular direction, we need to allow "racism exists" a fair turn as the null hypothesis to see if the data can reject it.
I will come back to how this might be done in a minute. Before I do, some readers might think that the presence of racism is so harmful that treating the two hypotheses -- "no racism" and "racism exists" -- symmetrically is not what we should do either. Instead, the presumption should be that racism exists unless there is overwhelming evidence against it. In this case, we do not need two tests as in the non-nested model comparison example, but rather we need a single test where the null hypothesis is "racism exists" and "no racism" is relegated to the alternative hypothesis.
There seems to be much merit in this idea. Surprisingly, it reminds me of time series econometrics.
Honestly. In time series, there is nothing more important than knowing whether the data are stationary or nonstationary. If the data are nonstationary and this is ignored, all hell breaks loose. Because ignoring nonstationarity is so disastrous, the majority of statistical tests frame the test by defining the null hypothesis as nonstationarity and the alternative hypothesis as stationarity. The rationale is that unless the data provide overwhelming evidence of stationarity, one should err on the side of failing to reject nonstationarity.
If nonstationarity is that important, clearly racism merits the same consideration. We should err on the side of failing to reject the null hypothesis of "racism exists" unless we find overwhelming evidence to the contrary. This calls for flipping the null and alternative hypotheses, as suggested by Mar.
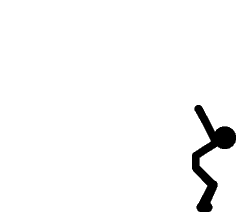
Of course, the argument laid out here applies to any use of NHST, not just racism. Researchers should be more aware of the implicit bias that is created by the choice of which hypothesis to assign as the null and which as the alternative. We need to avoid framing the test in a manner that biases us toward a particular answer, especially if that answer coincides with our prior or makes our lives easier.
As mentioned above, a final comment is in order. I saw several replies to Mar's thread stating that it is not obvious how one could flip the null and alternative hypotheses in the context of testing for the presence of racism. The difficulty arises because the hypothesis of "no racism" typically lends itself to a point in the parameter space of one's model, while the hypothesis of “racism exists" can manifest itself in an infinite number of ways.
In NHST terminology, "racism exists" is a compound hypothesis, while "no racism" is a point hypothesis. And, NHST requires a point null hypothesis.
This is true, but I have three responses. First, let's not settle for a test that is biased in the direction of not detecting racism just because fixing the issue may be difficult. We have solved far more difficult problems, I'm sure. Second, again, time series may provide some insight to a solution.
In the case of testing for stationarity, the hypothesis of nonstationarity also encompasses a vast majority of the parameter space; nonstationarity is a composite hyopthesis. However, the null hypothesis is specified as the edge of this space. In time series jargon, a unit root is the least favorable case in which the data are nonstationary. Depending on the model being estimated, one can surely devise a way to frame the null hypothesis as corresponding to the least favorable case in which racism exists.
Third, a recent paper by Masten & Poirier (2020) builds on the logic of the least favorable case and devises an alternative way of conducting hypothesis testing. This new approach could be extremely useful in the context of testing for the presence of racism. Instead of maintaining a set of assumptions, obtaining an estimate of the model parameters under these assumptions, and then conducting NHST, the authors propose specifying a particular hypothesis and then finding the minimal set of assumptions consistent with the hypothesis. The complete set of assumptions consistent with the hypothesis is referred to as the breakdown frontier. The authors write:
"Given a set of baseline assumptions, a breakdown frontier is the boundary between the set of assumptions which lead to a specific conclusion and those which do not."
As Masten & Poirier (2020) propose and illustrate this approach within the potential outcomes framework and hypotheses concerning the average treatment effect, this method seems ideally suited to hypotheses related to the presence and extent of racism.
I hope our world is changing. We all can and must do better. While a teeny drop in the bucket, thinking about how we frame and conduct our hypothesis testing is one such way.
References
Kennedy-Shaffer, L. (2019), "Before p<0.05 to Beyond p<0.05: Using History
to Contextualize p-Values and Significance Testing," The American Statistician, 73(sup1), 82-90
Kuffner, T.A. and S.G. Walker (2019), "Why are p-Values Controversial?," The American Statistician, 73(1), 1-3
Masten, M.A. and A. Poirier (2020), "Inference on Breakdown Frontiers," Quantitative Economics, 11(1), 41-111